ITMO Researchers Predict Carbon Particle Properties for Cancer Diagnostics
A group of researchers at ITMO has developed a new machine learning-based approach to producing carbon nanoparticles for biomedicine. Thanks to the algorithm, scientists will be able to select the necessary parameters for synthesis of carbon particles with target properties. In the future, the particles can be used for biovisualization of tumors and their treatment with phototherapy. The new approach is described in a paper published in Small.
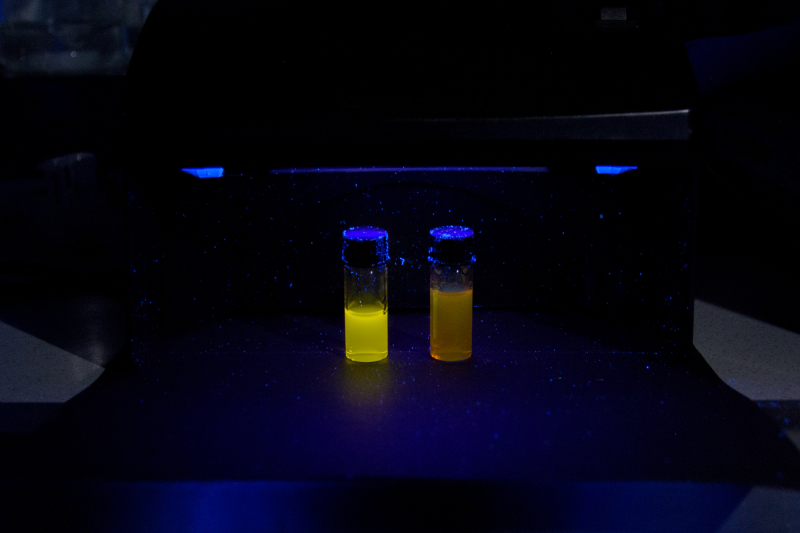
Nanoparticles find one of their applications in cancer treatment and diagnostics – they help detect tumors during MRI and luminescence microscopy. Carbon nanoparticles are a good candidate for such contrast agents: they are easy to create, biocompatible (i.e. not harmful to the human body), and their properties can be controlled. Those particles that can be used in treatment and diagnostics have to radiate light in the red band of the spectrum, where tissues are transparent and easy to visualize. Usually, such particles are developed by trial and error: scientists synthesize and test particles with various parameters, however this approach isn’t always efficient and is time-consuming.
Researchers from ITMO have suggested automating the selection of carbon nanoparticles and developed a machine learning-based algorithm. As its input, the model takes particle synthesis parameters (type of precursors, molar ratio, solvent volume, synthesis time and temperature); as the output, the algorithm predicts the optical properties of nanoparticles: absorption and photoluminescence peaks, as well as quantum yield of photoluminescence. Thanks to this, the researchers will be able to make the production of carbon nanoparticles with target optical properties for medical applications quicker.
Around 130 open-access results of carbon particle synthesis were used to train the model. Then, the algorithm was tested at three laboratories around the world: in St. Petersburg, Changchun, and Hong Kong, during a total of six syntheses. These experiments have shown that the model can efficiently predict the optical properties of nanoparticles: the efficiency metrics (coefficient of determination) reached 0.72 for syntheses with the same precursor and 0.86 for syntheses formed by the algorithm (the maximum value being 1).
“Even though the model currently works on a relatively small database, it is still the largest one for carbon nanoparticles. Our algorithm is openly available on GitHub for other researchers to use in testing different nanoparticles and expanding the database. In the future, we’d like to turn the model into a more general-use tool that can be applied not only for carbon nanoparticles, but for other cases where a link has to be identified between synthesis parameters and properties of particles,” shares Elena Ushakova, one of the paper’s authors, a senior researcher at the International Research and Educational Center for Physics of Nanostructures.
Onward, it will be possible to use the carbon nanoparticles synthesized with the algorithm for cancer diagnostics: for this, the particles will first have to be introduced into tissues and then analyzed using luminescence microscopy. Moreover, the nanoparticles with target properties can be used in photothermal cancer therapy; in this case, the particles will absorb light and turn it into heat, locally destroying tumors.
The project is supported by the Russian Science Foundation.