Predicting toxicity of chemical compounds
In order for any new drug to be safe, its contents must first be tested for toxicity. This characteristic can be manifested as mutagenicity, cancerogenicity, chemical contamination, liver damage, and so on. All of these can be predicted using the simplified molecular-input line-entry system (SMILES), which creates descriptions of molecular structures. SMILES helps produce graphs, language models, or descriptions of chemical properties to predict compound toxicity.
In this venture, as in many fields, AI comes to the rescue. For instance, with the platform Sintelli chemists can predict the physical, chemical, biological, and toxicological properties of organic materials, as well as visualize arrays of chemical data.
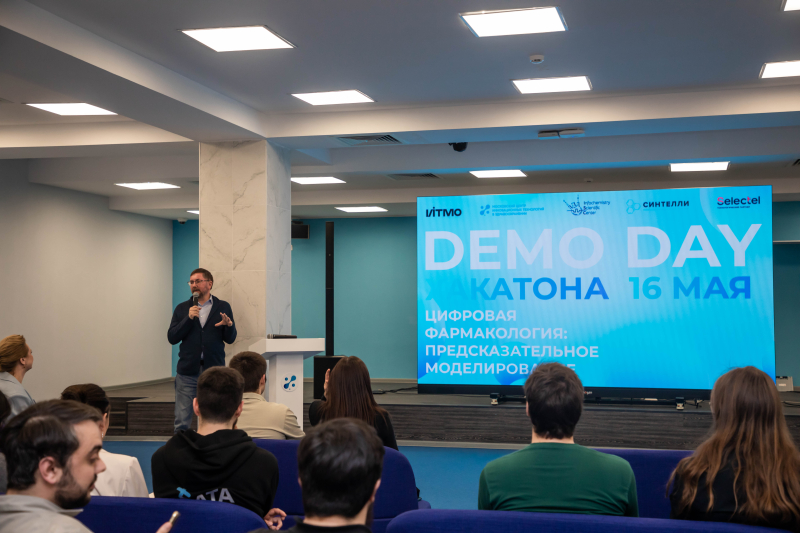
Digital Pharmacology Hackathon. Credit: Medtech.Moscow
Where’s the catch?
According to Maxim Fedorov, PhD, a corresponding member of the Russian Academy of Sciences and a co-founder of Sintelli, researchers have currently cataloged the toxicity of about 200,000-300,000 chemical compounds out of the nearly 200 million known to science (and the number keeps growing).
That’s why, for this hackathon, the participants were given the task of creating a machine learning model that would minimize the amount of time and resources needed to develop and implement new drugs – as well as limit animal testing and risks of negative side effects.
The 112 participating students spent 48 hours developing their solutions – 27 different algorithms. The three winning teams shared the 1-million-ruble prize and received invitations to enter ITMO’s online Master’s program Chemistry Software. They also have the opportunity to join the team of Sintelli and other medical startups. The event was held with support from Selectel.
“This hackathon is a chance for any student or young researcher to work on a real-world case in an interdisciplinary team at the intersection of chemistry, pharmaceuticals, and IT. For participating companies, this event is a chance to scout for new solutions. Their performance in the hackathon can help students enroll into our Master’s programs or land a job with one of our partners. These opportunities serve as additional motivation to use infochemistry solutions for research and medicine. By applying IT tools in chemistry, we can develop new drugs at a lower cost,” says Professor Ekaterina Skorb, the head of ITMO’s Infochemistry Scientific Center.
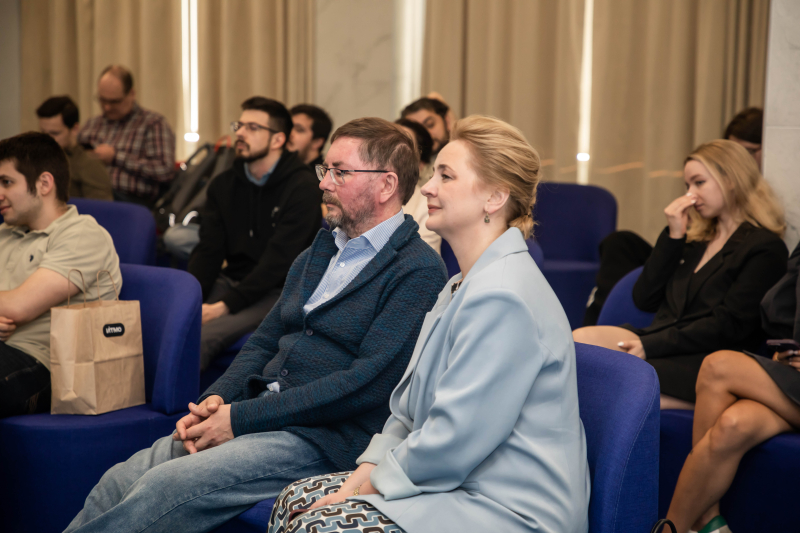
Ekaterina Skorb. Credit: Medtech.Moscow
Read also:
AI Plus Chemistry: Lab Robots, Drug Development and More
ITMO Scientists Develop Algorithm to Predict Nanomaterials Toxicity
Here’s what the participants came up with
Compiling indicators into a database and boosting decision trees. The first place was awarded to the team MML, which is composed of Moscow Institute of Physics and Technology students Nikolay Kutuzov and Sergey Novikov. They have developed a machine learning model that is capable of predicting up to 34 indicators of toxicity in chemical compounds. This model is based on a dataset of more than 1,100,000 SMILES molecule descriptions from 20 different sources.
“We came up with a simple and efficient way of compiling lots of data on molecules into a single set in order to then feed it into a machine learning algorithm. We used several types of indicators: chemical ones (such as a number of functional groups in a compound); those which came from language models that were trained on chemical formulae; mathematical ones that were the result of using graphs to represent molecules. Then, we turned to the machine learning model CatBoost – an algorithm designed for classification and regression analysis that is based on gradient boosting of decision trees. Such algorithms create a prediction in the form of an ensemble of weak prediction models,” shared Nikolay Kutuzov, the captain of the team.
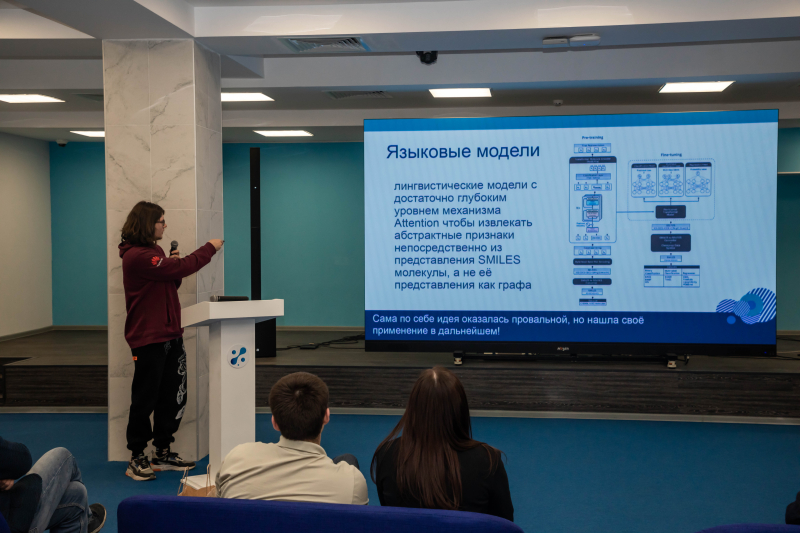
Nikolay Kutuzov’s presentation at the hackathon. Credit: Medtech.Moscow
This solution will be helpful to chemists, as it would reduce the number of compounds that need to be put through trials when developing new drugs. Another possible application lies in chemoinformatics, where this approach will increase the quality of automated drug manufacturing.
Developing multimodels that predict toxicity values. The second place went to the Billy QSAR team, which is composed of Ruslan Lukin, a team lead at Innopolis Univervity’s Machine Learning for Materials lab, and Boris Piakilla, a student at the Tomsk Polytechnic University. They have used CatBoost to analyze more than 84,000 molecules from 12 sources in order to develop multimodels that can predict 41 different indicators of toxicity.
As pointed out by Ruslan Lukin, this approach utilizes atom attribution, which helps interpret various toxicity parameters. This technique shows how each individual atom in a molecule impacts the overall prediction. First, the model takes a molecule and calculates its properties, such as its energy or thermodynamic activity. Then, the model predicts the properties of a similar molecule that is missing one of the original’s atoms. By comparing the two predictions, it figures out how each atom affects the molecule. The resulting impact is then visualized via graphs or 3D models.
This solution will be useful to pharmaceutical companies and R&D laboratories, which could use it to design molecules with the desired toxicological properties. It also could create models that can be interpreted via QSAR (quantitative structure-activity relationship – Ed.), so as to let other specialists predict their properties.
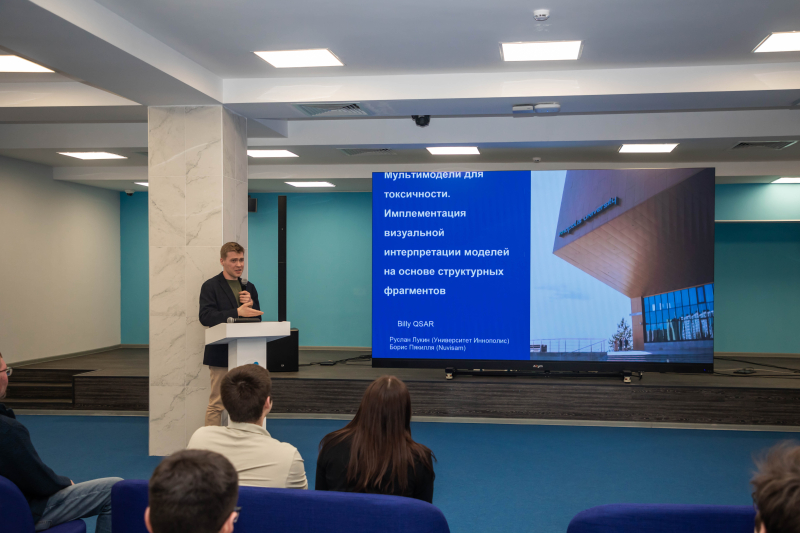
Team Billy QSAR presents their solution at the hackathon. Credit: Medtech.Moscow
Multi-tasking done right. The third place was taken by the team SCD Lab: Ivan Pikulin, Mikhail Rudenko, and Vladislav Yaryshev from Lomonosov Moscow State University; Anastasiia Yudina from the Russian State University for the Humanities.
Their solution is based on graph neural networks and classic machine learning algorithms. Having collected data about 78 molecules from six open sources, the students used it to train models that predict compound toxicity based exclusively on molecule structure formulas. According to Vladislav Yaryshev, their best models have an error rate of 10%, which is a good result for machine learning and pharmacology. Such models will already be helpful in filtering out the most toxic molecules at the early stages. A special feature of this solution is the use of multi-task learning for the graph neural network: it considers unapparent correlations between different properties of the same molecule. Thanks to this feature the model can identify such correlations on its own during training.
A potential application for this solution is drug development and, in particular, evaluation of the toxicity of potential active components.
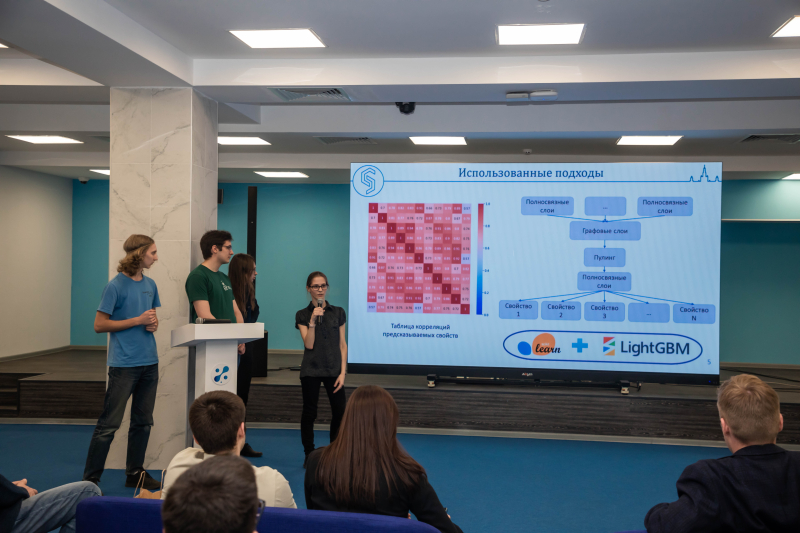
Team SCD Lab presents their solution at the hackathon. Credit: Medtech.Moscow