Antibiotics are used to treat bacterial infections, cure illnesses in humans and animals, develop medications and vaccines, protect crops and trees, and extend the shelf life of products. However, overuse of antibiotics leads to antibiotic resistance, meaning that bacteria grow to be insensitive to the treatment, which was once efficient.
A promising alternative to antibiotics could be nanoparticles based on silver, gold, zinc oxide, or copper oxide. These nanoparticles can inhibit the growth of antibiotic-resistant bacteria and kill the infection, but along the way they also destroy other microorganisms that are vital for digestion and the immune system.
Traditional synthesizing and testing of nanomaterials takes a lot of time and effort; and with this method, it isn’t possible to determine the selective toxicity of nanoparticles, which is their ability to destroy harmful bacteria without affecting benign ones. One reason is that the correlation between the parameters of nanoparticles (their shape, size, and composition) and their selective toxicity is yet unknown. To tackle this problem, scientists from ITMO’s ChemBio Cluster utilized AI and machine learning tools.
“We developed a screening platform to identify nanoparticles that selectively target and destroy pathogenic bacteria, such as those that cause pneumonia, while leaving “good” microorganisms untouched. The method will be beneficial in treating all antibiotic-resistant illnesses and might become a new strategy to fight diseases that can no longer be cured with current medications. These nanoparticles can find their use in both medicine and agriculture; they will help protect crops and livestock from drug-resistant bacteria,” explains Susan Jyakhwo, the first author of the paper and a third-year student at ITMO’s ChemBio Cluster.
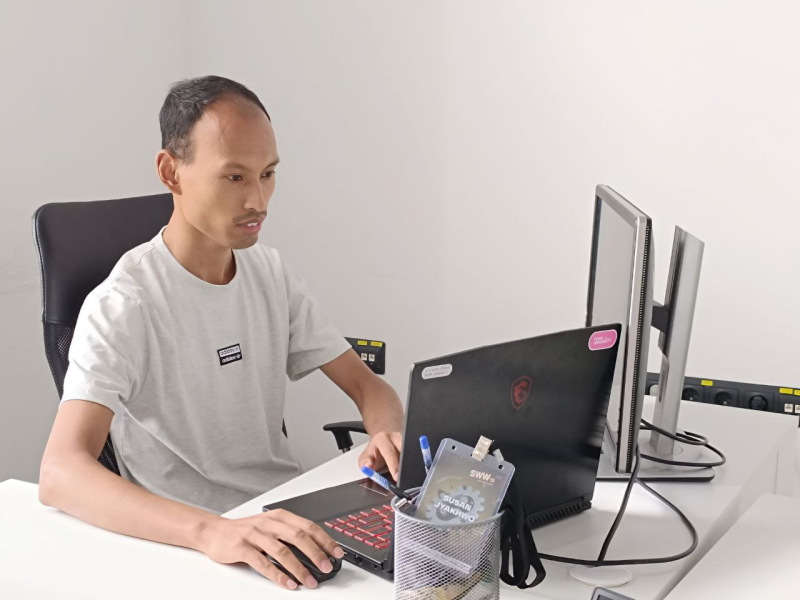
Susan Jyakhwo. Photo courtesy of the subject
The platform is based on a database of approximately 2,000 samples from over 70 research papers, including on physicochemical properties of nanoparticles, biochemical parameters of bacteria, and experimental findings. All data was examined using machine learning and a custom genetic algorithm. The latter is based on natural selection and genetics principles: it selects nanoparticles, combines their parameters, and chooses the best combinations. The algorithm analyzed over 500 samples within a second; the best candidates are being tested in the laboratory.
The platform helped researchers identify that copper oxide-based nanoparticles selectively destroy the pathogenic bacteria Staphylococcus aureus, while zinc oxide nanoparticles perform the same for Klebsiella pneumoniae. At the same time, both nanoparticles did not affect the non-pathogenic bacteria Bacillus subtilis.
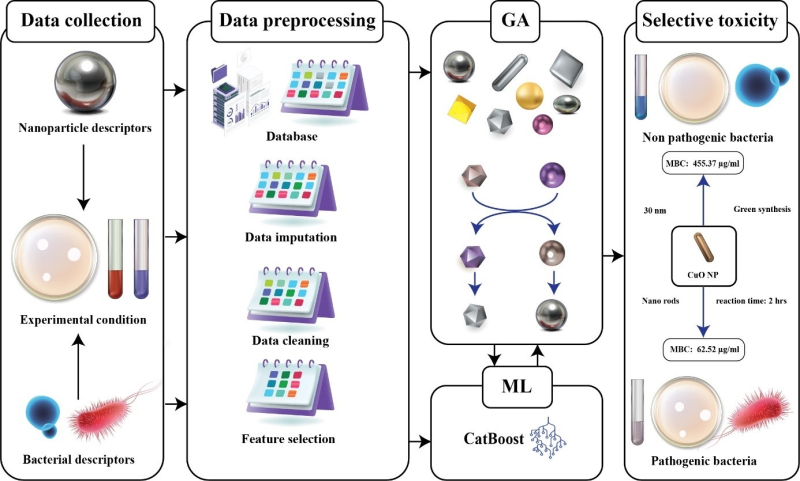
Selectively antimicrobial nanoparticles. Photo courtesy of the researchers
In the future, the researchers plan to shortlist selectively toxic nanoparticles that could be paired with antibiotic drugs for greater efficiency against infections. Additionally, selective nanomaterials may be employed for disease diagnostics, testing and development of biosensors. This invention will also improve the speed and accuracy of pathogen detection in both patient samples and foods. In the meantime, scientists need to assess the toxicity and immunogenicity (their ability to provoke an immune response in a human body) of selective nanoparticles in vitro and in vivo.
The research was carried out as part of the Priority 2030 program of the Russian Ministry of Science and Higher Education.