Complex systems
The word “complexity” comes from the Latin “complexionem”, which may be translated as “interconnected” or “tangled”; it refers, thus, to the interwovenness of various elements. Even more, there are important relations established between them. Take the atoms of carbon, as an example. Depending on their position relative to each other, they present different properties and form different objects. A diamond and a piece of chalk contain the same atoms, just differently structured.
These microscopic properties are the product of a process called immersion. The characteristics of immersion seem enigmatic to many, and that is because the information produced by the interaction of elements cannot be found in these elements.
Imagine you have a childhood friend named Pasha. You know everything about him: his favorite food, favorite music, his habits. All in all, you can almost perfectly predict his behavior in any situation. But then Pasha goes to study at ITMO University where he meets and falls in love with Masha. And now we see his behavior change. All of a sudden he begins to take better care of himself. The only way to understand the new Pasha is to take into account his newfound relationship.
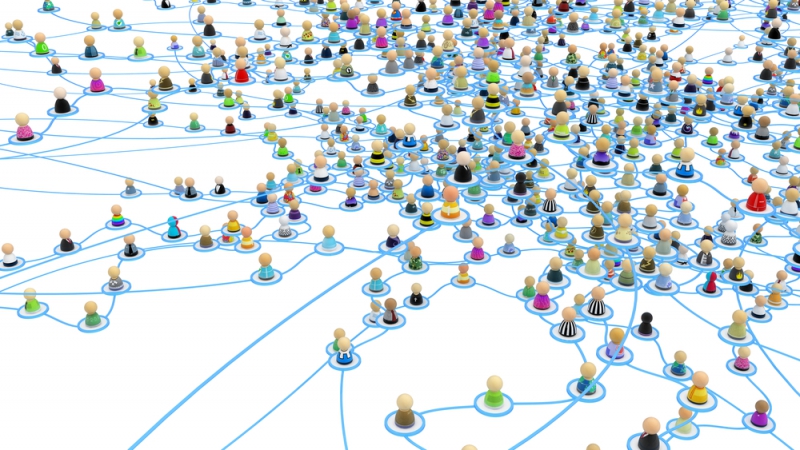
Years later, the couple has a child named Sasha. Their interactions with the child do not only generate new information about them, but also change the nature of the parents’ relationship.
Information, a very important consequence of an interaction, is not available immediately. In school, we learn from physics that by knowing the initial state of a system, we can predict its future. In the case of complex system, our ability to predict the future is severely limited; after all, we know nothing about the future interactions that are likely to affect the system.
The principle of reductionism
Science has traditionally adhered to the principle of reductionism: scientists would simplify phenomena in order to be able to predict them in the future. And that approach was successful: we built computers and found cures for many illnesses. As some researchers claim, humanity has already passed its epistemological period; nowadays, people die more often because of chronic illnesses. Unlike infectious diseases, chronic ones are more complex and multifactored. We still haven’t found a cure for flu, AIDS, cancer, diabetes, or heart disease.
And we can’t do that precisely because the reductionist method is useless in these cases: the systems are too complex and cannot be simplified due to the variety of factors and constants. For a while now, medical specialists have been promoting the concept of personalized medicine, wherein patients are thoroughly examined and given a selection of specific drugs instead of one common drug for all.
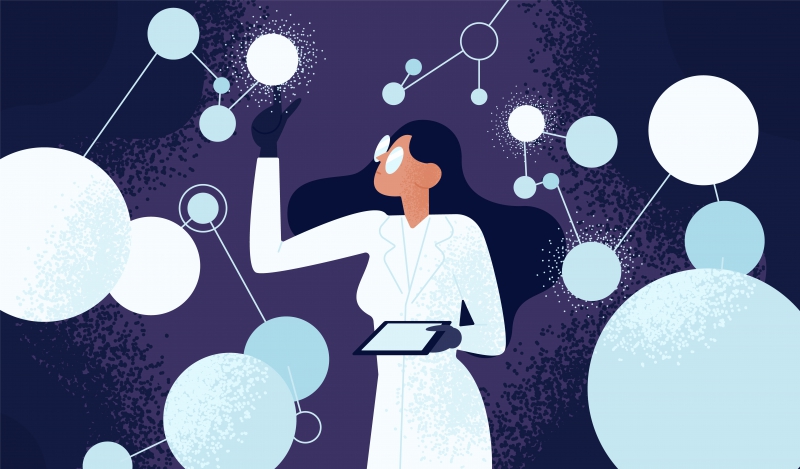
To understand the influence of complex systems analysis on modern science, we need only recall how research was done before. Scientists aimed to establish the laws of a phenomena and predict future. It’s almost like Laplace's demon: with a high enough level of intelligence, we’d be able to predict the entire past and future of the universe.
But that just won’t happen. Firstly, because of thermodynamics. We know that even with a large amount of information, we’d not be able to travel into the past because of the dissipation of energy. Many varied states all lead to one state. For instance, a group of people are present in this room, but being here does not define how we came here: by foot, on a bicycle, or by car.
It is the same with understanding complex systems: their components have all experienced a different past. According to the laws of thermodynamics, information is lost with time, and with it our ability to learn the past.
Why study complex systems now?
Our ancestors surely understood the complexity of processes and the importance of multifactority. For instance, Charles Darwin wrote in his On the Origin of Species that he was astounded by the complexity of a single ecosystem and the different species’ interactions with each other. But back then people did not have the necessary tools for in-depth analysis.
In the late ‘80s computers changed everything: they became our telescopes with which we studied complex systems. How did we study space before the telescope? It was impossible to see past the moon. It was Galileo Galilei who managed to see the moons of Jupiter with his primitive telescope. After that, our study of space has expanded far beyond our own limits of perception. Less than a century ago telescopes became powerful enough to let us learn of the existence of other galaxies.
It’s the same with computers. Before, we simply did not have the means to study processes with hundreds of thousands of variables. We could use math to solve differential equations, but the more variables they contain, the more difficult it is.
Computers give us the ability to understand how various interactions lead to various behaviors. Their computational capacity can handle complex systems with a million components. One program, Golly, lets users build a cellular automata, which is a grid filled with living or dead cells. As they interact with each other within the grid, they change. That’s why they also call it the Game of Life.
Since we cannot predict the past or future of complex systems, we must approach them differently. Perhaps in the future we would create an adaptive system that will take into account if not all, but many possibilities.