AI and the “machines of loving grace”
Machine learning is among the most accessible technologies related to AI. It’s been researched the most and offers a wide variety of instruments. Artificial intelligence is the ability of intelligent computer systems to perform creative functions that are believed to be the domain of human beings. It was back in 1956 that people first started talking about such “machines of loving grace”.
Starting in the 1980s, the field of AI has employed a statistical approach based on children's learning behaviors. For example, when a child writes their first text message, they only learn to print and pay no attention to mistakes. Children are great at enhancing their performance: they focus on communication only in order to get in touch. The idea is to teach a computer to perceive the real world as not a set of rules (as it usually does) but to build on how the real world perceives and uses data.
The advances we now see in the field of artificial intelligence became possible thanks to a change in both the approach and the overall conditions. The latter would be the emergence of cloud technologies (availability of huge amounts of data that can be used by AIs to learn), inclusion, and sensors (data entry points for AI) that are becoming more accessible.
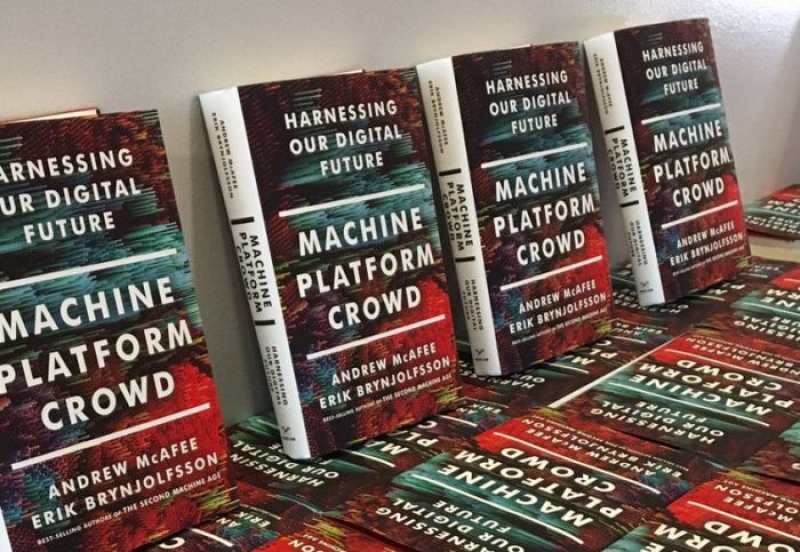
Andrew McAfee and Erik Brynjolfsson, the authors of Machine, Platform, Crowd, speak of an essential change: the transition from “standard partnership” to a new type of a relationship between humans and machines. The standard partnership is about machines, robots and algorithms carrying out tasks that humans don’t want to or can’t do for whatever reason. The new partnership implies that AI also performs functions that once were the domain of humans, like intuition and decision making. It turns out that in many instances, especially when there is insufficient data, the risks associated with the human factor or where a human would have to keep too many variables in mind, computers can offer better judgment. If machine learning is a technology that still exists within the context of standard partnership, most other AI technologies stretch far into the new concept of human-machine relationship, in which technology makes decisions that are believed to be better than those made by human beings.
Machine learning is mostly used for automating the tasks performed by humans: identifying patterns, diagnostics, classification, forecasting, recommendation, and image, speech and text recognition (many of these fields overlap). These are also the essential conditions for the more intelligent machines to learn and better comprehend reality.
One good example of the emergence of such technology is the Belorussian agricultural startup OneSoil that makes use of Big Data and machine learning methods to develop applications and an online platform for precision agriculture. They analyze airborne imagery and identify what grows on various fields based on data from the already examined ones. For instance, they’ve learned that there is a region in Estonia bordering the Leningrad Oblast where only grass grows.
In Russia, machine learning is used in facial recognition systems. Prior to the FIFA World Cup in Russia, Moscow Governor Sergey Sobyanin reported that law enforcement has apprehended their first criminal with the help of such a system. In the past two months, nine wanted criminals were also identified this way. The Government of St. Petersburg has also ordered 80 smart cameras that have facial recognition functions.
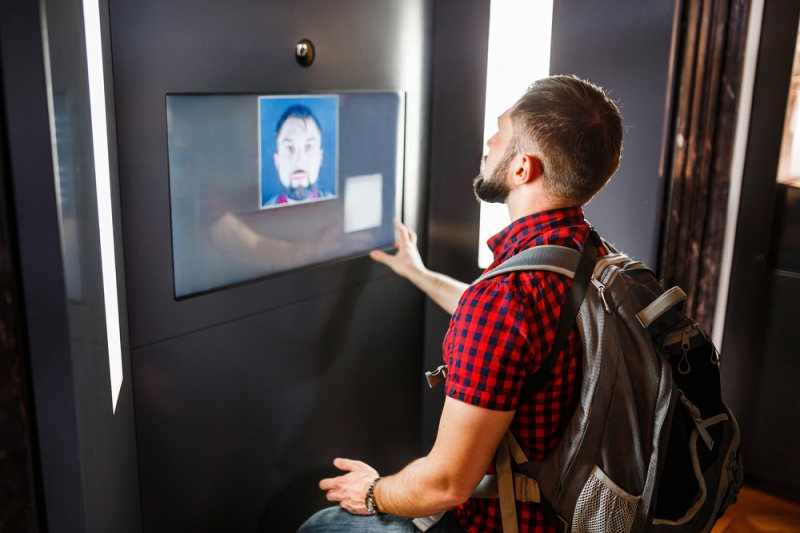
Still, the capabilities and ambitions of the “machines of loving grace” are far beyond that. Today, we have many examples of how AI can create portraits of seemingly real people in such a manner that you can actually believe that they could exist. There are also cases of AI creating images, composing music and writing poetry. Still, the problem here is that as long as such art is created by an AI, it won’t make much sense to people, as computers still can’t feel as we do. On the other hand, AIs don’t care whether we understand them or not.
An example of the new kind of human-machine relationship are Level 5 autonomous cars. When cars possess enough models to comprehend reality and enough information about the social realm, there can come a day when we won’t have to make decisions: first, we don’t have to decide on our route, and then we won’t even have to tell the car where we want to go, as it already knows it better.
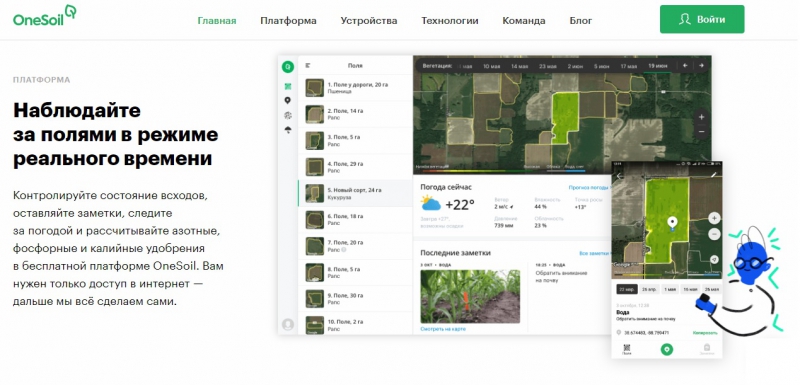
Issues associated with AI
Empathy issues
The issues related to empathy and the understanding of living beings is something that businesses and governmental agencies (i.e. those who have resources for developing AI) are less interested in, so they’ll be falling behind. So, robots will be unlikely to perceive our feelings as something of essence.
Ethical issues
Those who develop AI will endow it with the same prejudices they have; therefore, any biases a developer may have will have an effect on their creations. And the influence of machines and their algorithms is greater than that of particular people. You can have impudent neighbors, impudent politicians, but just imagine impudence from a system that affects everyone.
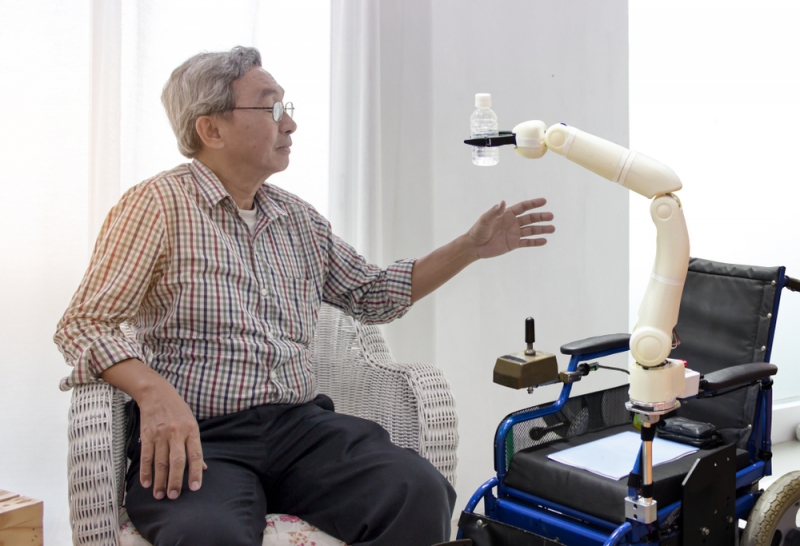
False correlations
A developer can easily tell how mistakes are made. But that mistakes and their complexity and occurrence rate grow with such speed that human beings can no longer control them. A good example of that is when a program that forms a queue for emergency patients puts asthmatic patients with pneumonia behind those who only have pneumonia. According to statistics, patients with asthma don’t die straight away, so the machine would decide that they have lower priority. In reality, such patients are to be treated first.
The “Black Box” problem
Machines that operate according to complex algorithms don’t store everything in their memory as regular computers do. The better the algorithms become (and you should mind that they constantly improve), the harder it is to decipher the “black box”, i.e. understand the essence of the algorithm and how exactly it makes its decisions.
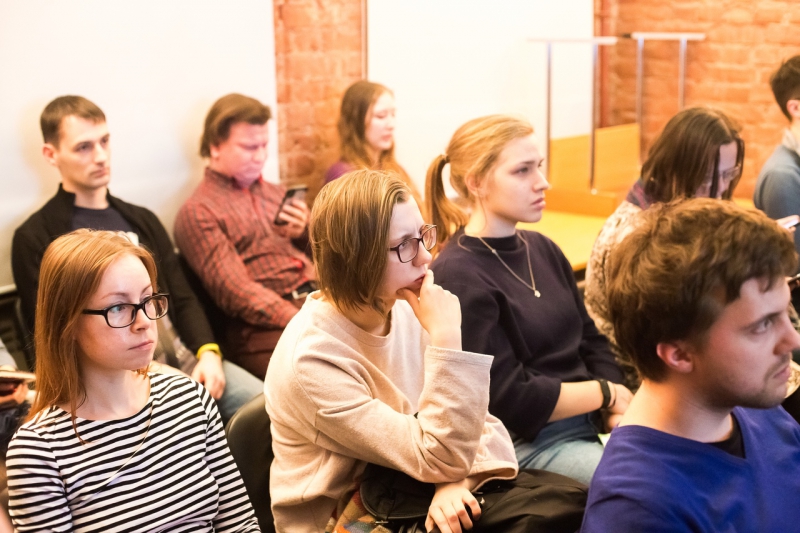
Due to the speed with which technology proliferates, and how it helps business and governments, people are interested in introducing it into as many areas of their lives as possible. For now, we don’t see the consequences of this process, but we can well compare this situation with that of other global technologies that were being developed with the best of intentions. The discovery of nuclear energy resulted in the concept of mutually assured destruction and the signing of nuclear non-proliferation treaties. Technologies take time to truly manifest themselves, and the mistakes we make today will affect our children and grandchildren.
Alexey Sidorenko founded the Greenhouse of Social Technologies educational project. Their team organizes hackathons and meetups in different Russian cities, oversees the Teploset online school, and develops free products for non-profit organizations.