About Cozzarelli Prize
The award was established in 2005 as the Paper of the Year Prize and was renamed in 2007 to honor the late PNAS Editor-in-Chief Nicholas R. Cozzarelli. Every year, the PNAS Editorial Board announces the list of the best research works honored by the prestigious prize.
This year, the judges chose 3,000 scientific research articles that appeared in the journal last year and represent the six broadly defined classes under which the National Academy of Sciences (NAS) is organized: physics and mathematics, biological sciences, engineering and applied sciences, biomedical sciences, behavioral and social sciences, applied biological, agricultural, and environmental sciences. The full list of the 2018 Cozzarelli Prize recipients is available here.
The 2018 awardees will be recognized at an awards ceremony during the NAS Annual Meeting on April 28, 2019, in Washington, DC.
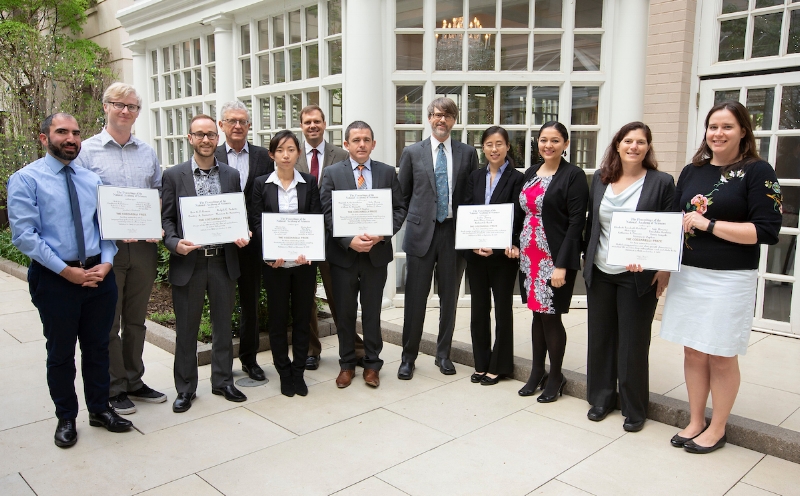
Machine learning for quantum experiments: how it works
Young Russian scientist Alexey Melnikov became one of the Prize awardees in physics and mathematics. He and his six colleagues received their award for their work “Active learning machine learns to create new quantum experiments”. In this article, researchers attempt to answer the question of how to accelerate discoveries in the field of quantum physics using machine learning technologies.
According to Alexey Melnikov, the main idea of the work is that we can delegate some of the most complex and difficult physical tasks to machines. One of such tasks is planning and design of quantum experiments conducted in optical laboratories, such as experiments with single photons, which are used for the creation of practical applications for quantum computations, quantum communications, and cryptography.
Such experiments are really difficult to conduct. Special equipment is used in quantum laboratories, while specialists’ task is to come up with the experiments’ design. As of now, this is (still) being done using standard approaches such as logic, experience and previously acquired results, estimation, and modeling. Specialists attempt to combine all these methods in a creative way to get an approach that would work. However, as technologies are gradually becoming more and more complex, this approach doesn’t work so well. In recent years, there has been a growing need for algorithms that would solve all these tasks and would be responsible for experiments’ design.
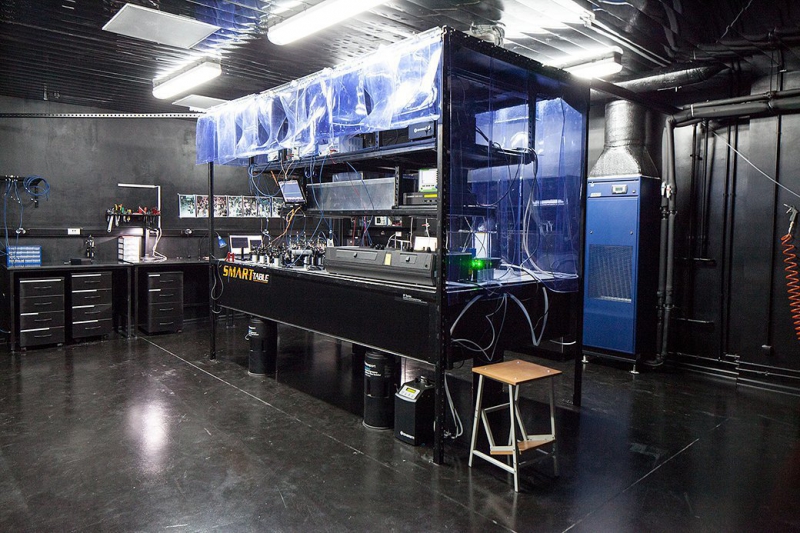
There have long been attempts to develop such an optimization algorithm, but they all reduced to simple enumeration of various opportunities in order to find something that would work, shares Alexey Melnikov. In his research, which he conducted together with his colleagues from the University of Innsbruck and the University of Vienna, Alexey Melnikov proposes a new method that allows the use of machine learning technologies for experiments’ design. Now it’s a machine that looks at the options and learning from its own mistakes helps to come up with the best solution. According to the results of the modeling, this approach is eight times more efficient than the previous optimization algorithms. However, increased efficiency is not the main advantage of the new method, the quality of experiments is.
“While all the previous approaches focused on finding a working solution, our approach is designed to find the simplest solution possible. Why is this important? Imagine that to solve a certain task you need a large optical table with lots of complex elements, which may result in a decrease in quality. Now imagine that you can solve the same task using a much smaller table, and you’ll get a better quality result,” comments Alexey Melnikov.
He adds that looking ahead, the scientists aim to continue and expand on their study. Many research groups have already started to apply similar approaches, which signifies of the prospects for the method’s further development, including in terms of its applications in other experiments in the field of quantum computations and quantum communications.
Research at ITMO University
At ITMO University, Alexey Melnikov works with the research group of Alexander Alodjants, a professor at the Faculty of Laser Photonics and Optoelectronics and leading research associate at the Laboratory of Quantum Cognition and Intelligent Systems. The scientist heads “Artificial cognitive systems based on quantum principles”, an interdisciplinary project implemented within the framework of the Project 5-100, and “Machine learning for hybrid quantum processing of data and metrology”, an international project supported by the Russian Foundation for Basic Research (RFBR).
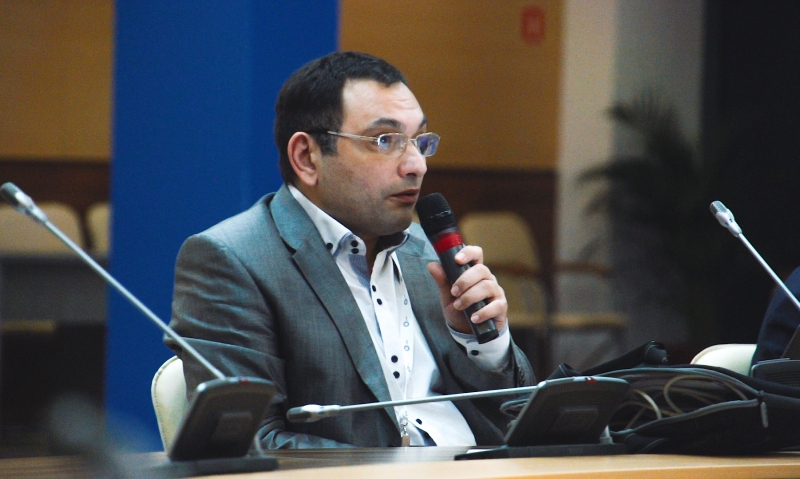
According to Alexander Alodjants, the main goal of the “Artificial cognitive systems based on quantum principles” project is the development of quantum algorithms for the searching of data, quantum transport in complexly structured systems and graphs, as well as their optimization based on machine learning methods. To that end, the scientists set two objectives to achieve.
The first is to develop a new applied information technology improving on the existing methods of the searching of data, semantic annotation and indexation, ranking of textual documents by means of quantum logic models applied in the modeling of human cognitive states, as well as to optimize the obtained results via machine learning methods. The second is to develop systems for the recognition of time complexity and high-speed response of specific classes of physical algorithms of quantum random walk on graphs based on machine learning methods. This last question falls into the remit of Alexey Melnikov’s work.
“The project will conclude this year, so the main results have more or less established themselves. Our main practical result comes in the form of a prototype of a data retrieval system operating combined methods and approaches to data processing as developed by us. Our main result in terms of theory, which is what Alexey and I have been working on, will be a system of learning and recognition of graph structures which demonstrate quantum supremacy in solving a variety of applied tasks. The very phrasing of this objective is completely unique and knows no existing analogs. Our system allows to forecast the effectiveness of computations on a quantum simulator (or even a quantum computer) with considerations of its specific architecture to a very high mathematical probability, thus allowing us to answer a key question of whether we could obtain the desired speedup effect if we use this or that quantum device,” comments Alexander Alodjants.
The results of the work have been published in the article “Detecting quantum speedup by quantum walk with convolutional neural networks”.
“In January, we completed the paper where we showed that machine intelligence learned and proved itself able to function as an export telling where to expert quantum speedup and where not. Imagine that you built an expensive installation (for example, a quantum simulator), but how to tell if there’s any use in it being quantum-based? This is a very important and topical question. For example, in the US, there is a range of companies that claim that they’ve created a quantum computer or, more than that, are already selling these devices. But it’s not clear if these computers are actually better than classical methods,” says Alexey Melnikov. “There is a paradigm of quantum speedup on graphs, but in reality, it’s really not that obvious: just looking at one graph can’t possibly enable us to give an on-the-fly ruling on whether there will be a speedup or not. Though some experts are capable of doing that, their intuition is not fault-proof. That’s why it would be great to have an automated system, an algorithm, that would unite expert knowledge in one. And we did in fact manage to make such an algorithm, to harness machine learning in a way to understand whether there would be a quantum speedup or not.”
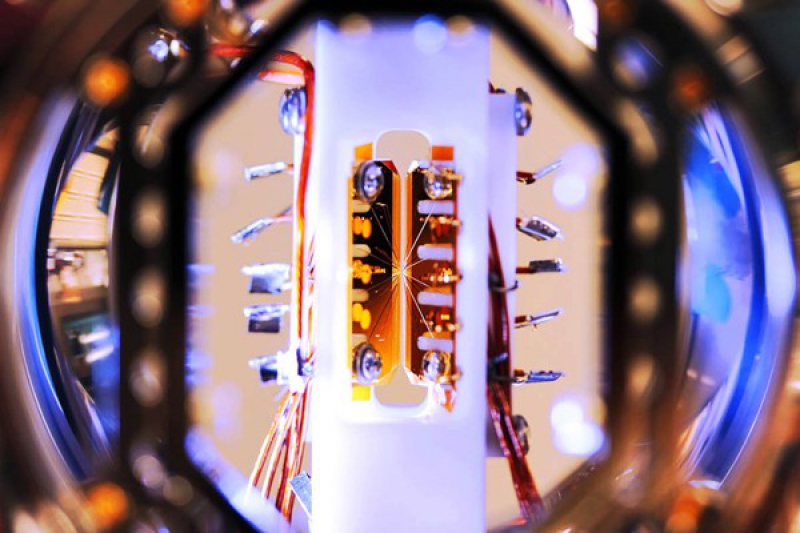
The researcher adds that in the near future, the group also plans to build on the results they achieved. Another project, “Machine learning for hybrid quantum processing of data and metrology”, implemented with support of the Russian Foundation for Basic Research (RFBR), is starting this year. Its key result is expected to be the development of smart sensors and sensors capable of working beyond the standard quantum limit of measurement sensitivity. The project will span three years.
“I’ve been working at ITMO University’s Laboratory of Quantum Cognition and Intelligent Systems since last year, though I did have some contact with my St. Petersburg colleagues prior to that as our research interests have a lot in common. What I like about working here is first and foremost the interesting research thematics. The laboratory specialists don’t spend their time solving routine engineering tasks but look towards the future; they’re interested in developing new and promising methods and approaches. The laboratory works in a wide, interdisciplinary field which, as I see it, has a lot of potential in terms of not only obtaining lots of qualitatively new, fascinating results but also achieving a variety of significant scientific breakthroughs,” concludes Alexey Melnikov.