The lecture was given as part of the Scicomm Cocoa Talk series by ITMO University’s Center for Science Communication. In this article, you can find the key ideas mentioned at the lecture.
Donald Campbell was a prominent American psychologist, sociologist, and methodologist. Throughout his scientific career, he was engaged in questions of social institutes’ assessment. In his key article, Assessing the Impact of Planned Social Change (1974), he formulated a principle which is nowadays known as Campbell’s law and often used in economics and politics.
As Campbell was observing social and bureaucratic institutes (including education, army, police, and so on), he noticed that the implementation of any criteria or indicators according to which the functioning of an institute was being evaluated unavoidably distorted both the indicators and processes in question.
Even though more than 40 years went by and management tools were mostly digitalized and algorithmized, Campbell’s law still works, disregarding all the efforts to overcome it.
Short-termism and working to improve the numbers
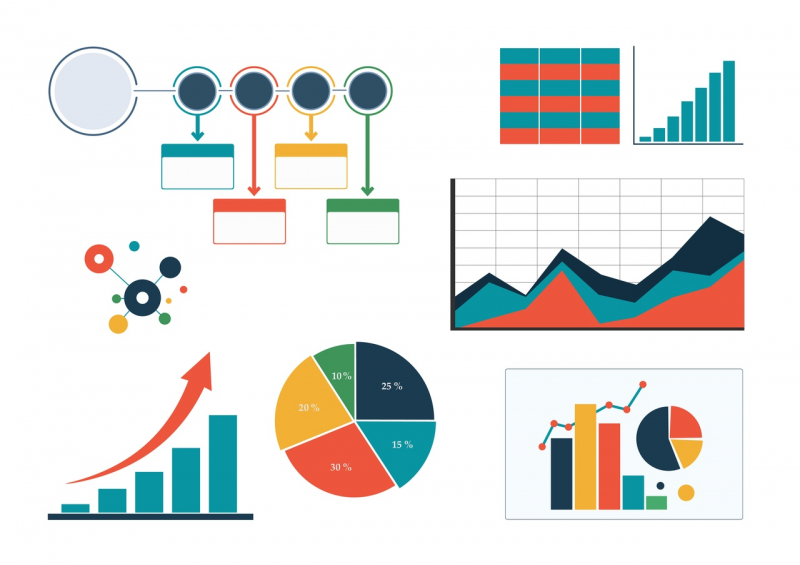
As any manager or official knows, it’s impossible to manage people or social institutes efficiently without some evaluation criteria or indicators. However, indicators aren’t just a tool for assessment but also a reason to decide whether people get rewarded or punished. So, once they are implemented, both the indicators and the processes they evaluate get distorted.
Donald Campbell used school as an example. Officials from the Ministry of Education want to understand how well the learning process in a school goes and how it can be improved. In order to achieve this, they use certain indicators. For example, average exam grades, the number of golden medals awarded, and so on. Whether a school will be rewarded or punished depends on these indicators. Basically, the life of this school’s teachers and administration depends on them.
In this situation, it’s highly possible that teachers will attempt to improve their results not by working on the level of teaching but, for example, by training school students to solve a certain exam pattern.
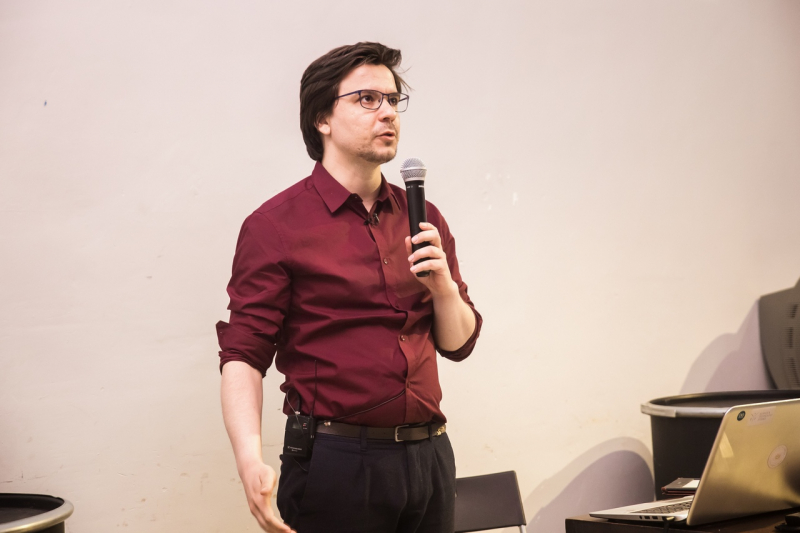
Another example is police. A certain amount of solved crimes is expected from the staff members, which leads them to solve minor crimes instead of complex ones – it makes reporting easier.
Modern researches expanded Campbell’s law and determined three strategies of social processes’ distortion: plain lying, fabrication of numbers, and focusing on the improvement of numbers while ignoring the rest of the work. It’s dangerous because of so-called short-termism – a phenomenon which occurs when global goals are ignored in order to achieve a lot of short-term ones. Another danger is oversimplification that neglects the complexity of processes.
Human factor and algorithm-based management
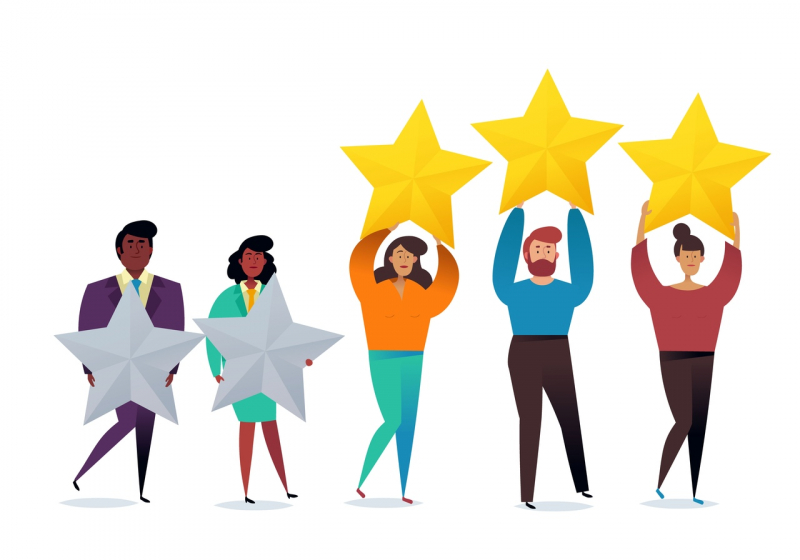
It may seem that all these distortions are caused by the human factor: people want to be rewarded and avoid punishment by the simplest means possible.
Initially, we thought that switching to digital tools – based on statistics, big data, and algorithms – will make the management better, more objective, and more efficient. However, for some reason, it didn’t.
The digitalization of police is a classic example. There are long-known problems in American police system: corruption and a high rate of discrimination towards certain races and towards people living in poor neighborhoods. Digital technologies were supposed to lessen these problems and prevent crimes more efficiently.
Sarah Brayne, an American sociologist, has been conducting large-scale research on the police of Los Angeles for two years. She accompanied police workers on raids and calls, observed the job of detectives, and, as a result, wrote a paper titled Big Data Surveillance: The Case of Policing. As part of it, she tries to understand whether implemented technologies like Operation LASER and Palantir had an impact on police work.
Operation LASER is a database and a ranking system. Each neighborhood and each citizen gets points. Members of armed groups and recidivists who repeatedly committed minor crimes get the maximum amount of five points.
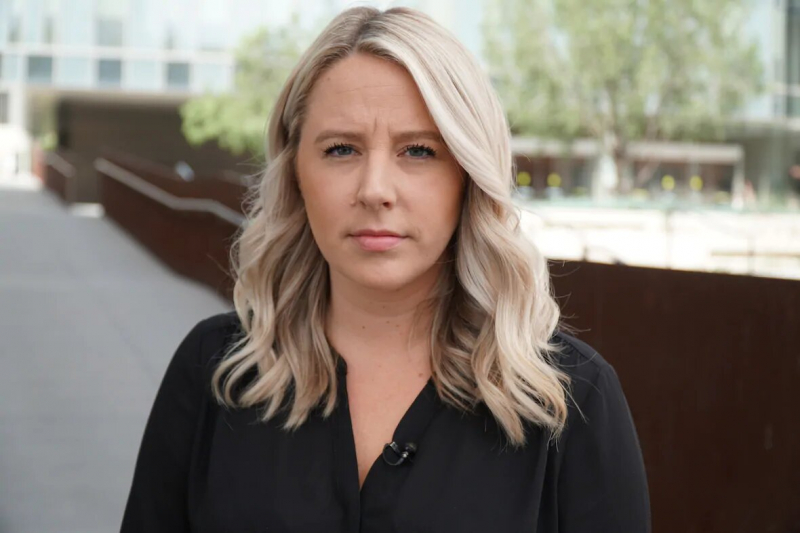
The problem is, one point was added for each time a person was stopped by police on the street, even if there was no specific reason. Police officers could stop anyone they wanted, for example, because of their suspicious appearance. The mere fact of filling out a contact card during such arrests had an impact on the person’s status in the database. The more points they got, the more often they were arrested – it was a vicious cycle. High ranking in the database allowed police to track this person, even though they didn’t commit any major crimes, or any crimes at all.
A similar problem occurred with the Palantir system. Palantir is a software based on data mining. It helps to see connections between a person – for example, a dangerous criminal – and property they owned, locations they went to, means of transportation they often used, people they contacted, and so on.
It means that people who didn’t commit any crimes appeared in the surveillance system because the algorithm decided so. Plus, friends and partners of criminals often belong to the same race or live in the same neighborhood, which increases the discrimination.
Algorithms in platform economy: how Uber drivers cheat
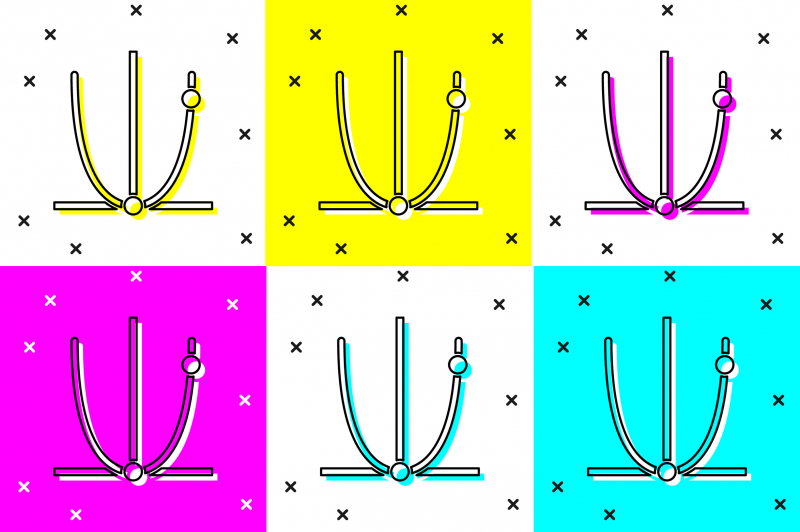
Campbell’s law also applies to commercial companies. Similar problems occur there.
Platform economy companies, such as taxi applications, food delivery services, and hotel aggregators like Booking.com and Airbnb, are closer to the solution of this problem. In these applications, management is performed by algorithms, while a small number of staff members only support it. This solution is mostly a necessity: one head office wouldn’t be able to manage millions of remote employees from all over the world.
Algorithm-based management was supposed to be a fairer, more objective and efficient tool due to the elimination of the human factor, but it didn’t turn out like that. Users quickly figured out the rules and learned how to bend them.
It can be illustrated by Uber. Initially, the person who works more was supposed to get more rewards. However, taxi drivers learned how to cheat: they left the driver mode on, parked near other Uber drivers, and allowed them to accept all the orders while doing nothing themselves. They also learned how to avoid unwanted passengers: they turned the driver mode off while driving through dangerous or suburban neighborhoods, avoided streets with lots of bars, and preferred to work on the streets with a lot of fashionable restaurants and hotels.
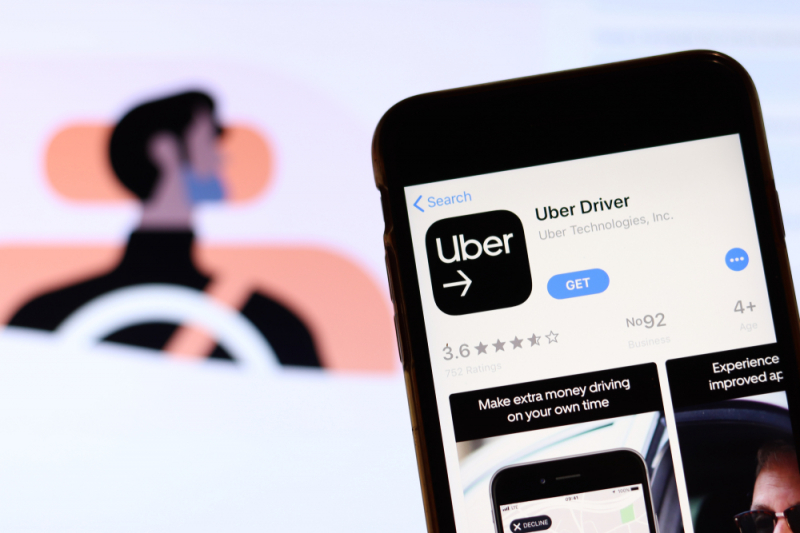
Researchers state that the reason behind this lies in the algorithm’s imperfection. It doesn’t see the difference between the passengers and neighborhoods. It also doesn’t take into account the features and differences that drivers have to face. That’s why users have to customize or bend the rules.
Some companies try to overcome the human factor by making up lots of vague indicators that users can’t figure out. That’s what they did at Airbnb: hosts didn’t know according to which criteria their offers appear higher or lower in search results. That’s why they were trying to make their services look better: they took nice pictures, set up fair prices, responded to all the clients, and tried to make their stay as comfortable as possible in order to get good reviews.
However, a research conducted by the company’s experts showed that they also got more anxious because they didn’t understand how the algorithm worked and what exactly it evaluated. People became more concerned with figuring out the algorithm rather than with increasing the quality of their services. The solution only created new problems.
Why Campbell’s law is so hard to overcome
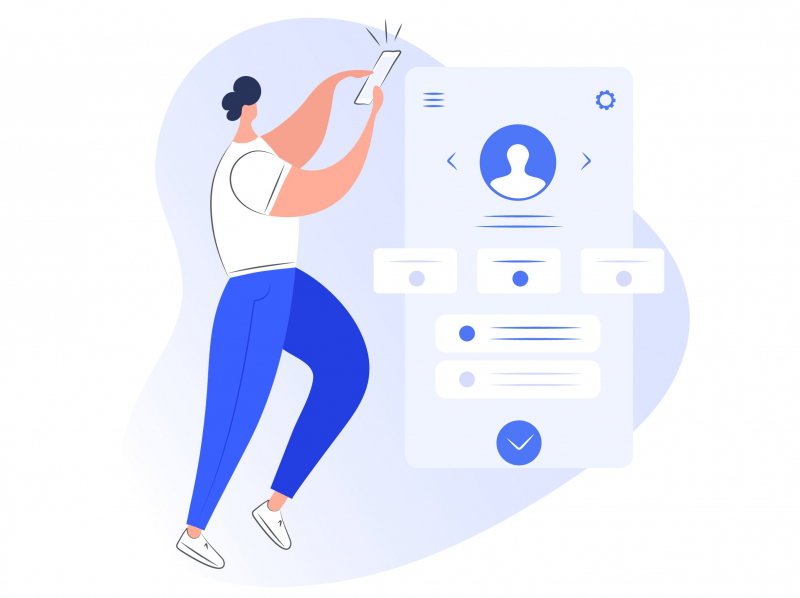
Donald Campbell suggested several strategies to deal with the law he discovered, but most of them are hard to follow. One of his suggestions was to not directly link the numbers and the rewards. However, in this case people mostly lose motivation to improve their indicators. He also suggested implementing lots of vague criteria, but the example of Airbnb proves that sometimes it only causes new problems.
Modern researchers believe that there are only two ways to minimize Campbell’s law: to research and observe not only the functioning of algorithms but also the user-algorithm interaction (science and technology studies, human-computer interaction, and user experience all deal with such problems), as well as to adapt the algorithms for the uncertainty of the environment and the features of human behavior.