According to Darya Chirva, head of ITMO’s Thinking module and curator of the project, the main aim of Hard Core Philosophy is to give an overview of the contemporary philosophical approach to such fundamental questions as the nature of cognition and free will without oversimplifying these questions or resorting to simple theoretic schemes.
“There are two tendencies in our contemporary perception of philosophy. The first one is to reduce it to a number of critical thinking methods. It is obvious that we have to think critically in our day and age, when we exist and make our decisions with an excess of information at our disposal and a variety of tools to manipulate opinions. It is crucial to have autonomous ways to navigate this world, evaluate any situation, detect fake news, and so on,” says Darya Chirva.
The second trend, according to Darya, is a growing general interest in philosophy with its unbiased view of the world. Philosophy can question the traditional methodological schemes in science. More and more specific issues, both theoretical and practical, are raised now, while brand new fields of research keep emerging, that we have yet no standards to work with.
“I am talking about, for instance, the question of the amount of influence that science can have on the social sphere, the possibility of replacing researchers with AI, the legal and ethical statuses of robots, digital versions of our personalities, and so on,” continues Darya. “Our project is meant to introduce researchers to those philosophical concepts that might be interesting and useful to develop ITMO’s scientific and technological projects.”
The first lecture was held by Dr. Alexander Gebharter, principal investigator at the Munich Center for Mathematical Philosophy at the Ludwig Maximilian University of Munich, and Dr. Christian Feldbacher-Escamilla, a research fellow at the Düsseldorf Center for Logic and Philosophy of Science at Heinrich Heine University Düsseldorf.
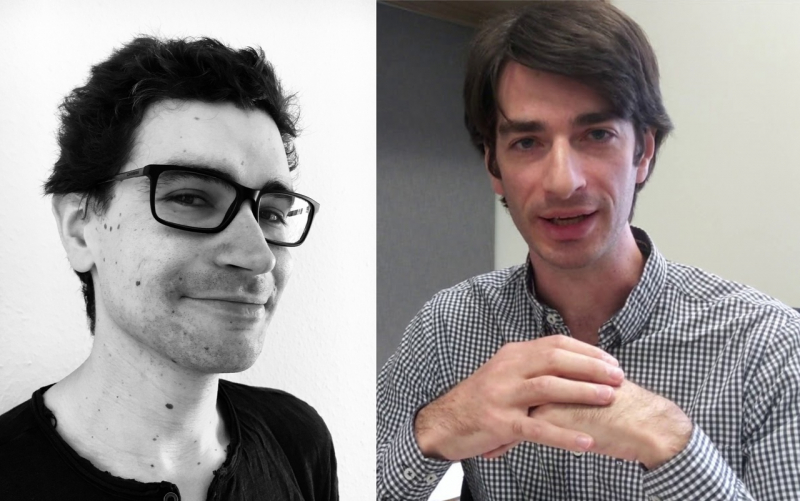
They talked about the methodology of decision-making in politics and management, the problems of randomized controlled trials (RCT), and unavoidable mistakes in RCT-based conclusions.
ITMO.NEWS has prepared the key points of the lecture titled “Causal inference in evidence-based policy. A tale of three monsters and how to chase them away”.
The three monsters of thinking
Making political decisions means efficient planning and forecasting. It is standard today for each strategy step to be supported by factual data – the more precise and verifiable, the better.
Increasingly often, politicians use the method of randomized controlled trials, but is it so accurate that you can rely on it unconditionally?
As practice shows, despite the fact that RCTs are based on correct causal relationships, these data are not sufficient to justify a particular policy, since they usually deal with only a small part of the overall causal structure and do not take the whole picture into account.
The speakers called the potential mistakes in identifying causal links “the three monsters” of evidence-based policy and named them P-Kong, Scylla and Charybdis.
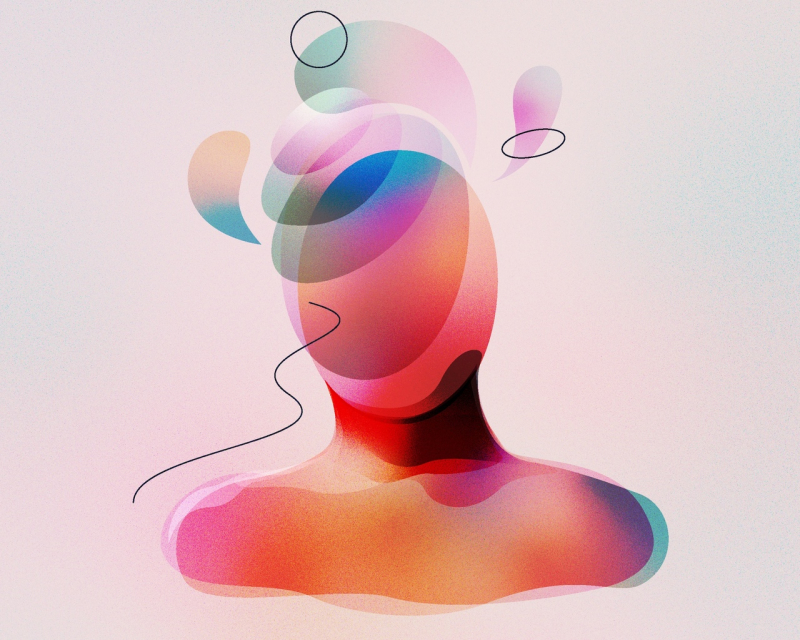
What kind of policy is proven to be efficient?
To begin with, it is necessary to state what an effective policy actually is. It seems to be intuitively clear: effective policies lead to the common good and improve the quality of life. But the speakers offered a more detailed definition.
First, a particular political decision should improve a situation, while not having significant undesirable side effects. Secondly, its implementation should not require inadequate costs and resources. And, thirdly, it must, of course, be approved by society.
How is the issue of efficiency viewed in science? The traditional decision-making theory distinguishes between two approaches: thinking-based or speculative and evidence-based. It should be noted that the first method is now considered obsolete, and all modern science is based on data and facts.
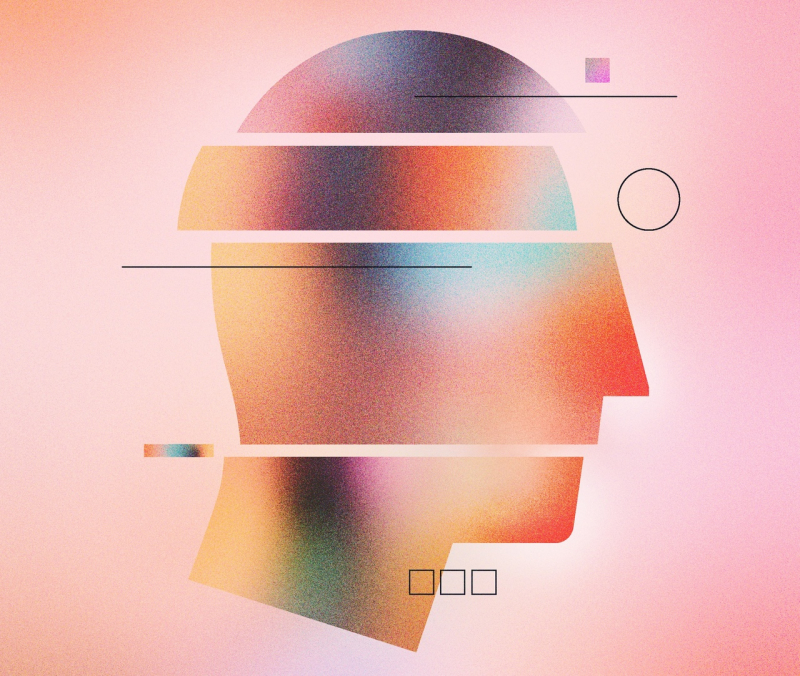
What’s wrong with randomized controlled trials?
Usually, RCTs and meta-studies (i.e. statistical analysis) are considered trustworthy for justifying the effectiveness of any decisions. Currently, RCTs are the golden standard in the field of effective political decision-making.
The following picture seems quite logical. We have two randomly formed groups. We applied a certain political decision to one of them and got the result we expected, while we applied nothing to the other group and saw that no changes occurred. This means that the solution is effective and well-founded, and therefore can be applied to other groups. But the underlying problem with the RCT method is that it is based on induction, that is, we extrapolate a particular case to the whole picture.
The evidence-based scheme of RCTs suggests that if the desired result is obtained in one area and with one category of people, then it will work for every other group. The randomization and the presence of a control group are supposed to ensure that this effect is not random and is not determined by the qualities of the subjects themselves.
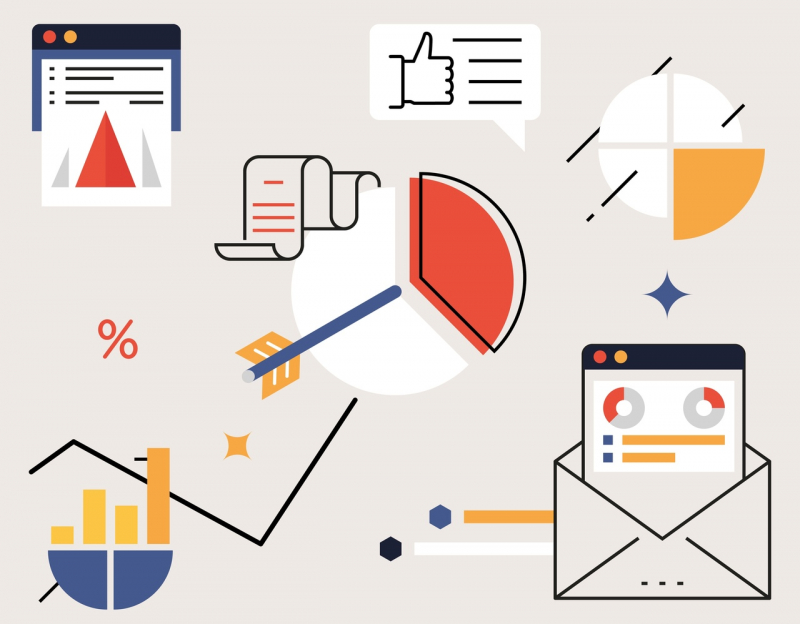
P-Kong and the problems of induction
It is here that we meet our first monster, Popper Kong or P-Kong. It is named after the famous and very influential philosopher of science Karl Popper. He not only founded critical rationalism and established the principle of falsifiability in science, but also became a prominent representative of postpositivism, which seriously criticizes the method of induction itself.
Popper considered it fundamentally wrong and claimed that we could only make truly right conclusions about anything – both in science and in politics – based on deduction.
This problem is discussed in detail by Jeremy Hardie and Nancy Cartwright in the work Evidence-Based Policy: A Practical Guide to Doing It Better.
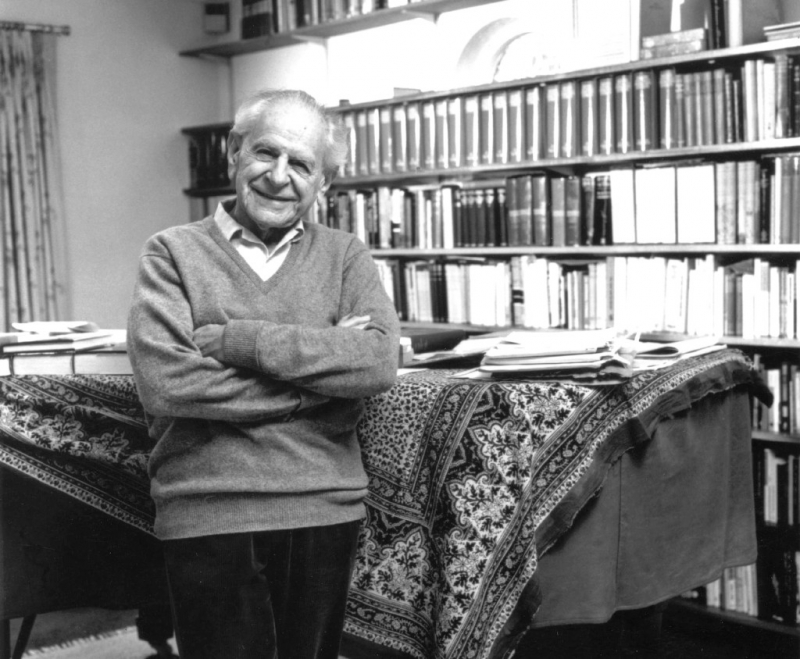
Their main idea is that if a political solution worked, for example, in one city or in one country, it does not follow that it will necessarily work in other cases. Because the positive effect could be influenced by a whole set of factors characteristic of a certain situation, which is completely ignored when we inductively generalize.
A political solution is just one ingredient in this pie recipe and there is no guarantee that when you add it, the pie will necessarily turn out delicious. Therefore, when evaluating success, you always need to take into account additional factors and causal relationships between the elements of the whole. The observed facts alone are not enough for us to justify a political decision. To avoid the problems caused by inductive inference, it is possible to apply a deductive scheme, which is what Cartwright and Hardie suggest that we do.
The Scylla and Charybdis of decision-making
Here we are inevitably faced with two new problems: we either know all those additional factors and the structure of causal relations of the new group in relation to which it is necessary to form a policy, or we don't.
In the first case, we meet the Scylla of deduction: if we already have the exact "recipe" and all the necessary components for a new group, then we do not need any example associated with another group in the theory of RCT. It is irrelevant. We know enough to be sure that our decision will be successful. Thus, the very idea of evidence-based policies (or RCTs) is discredited.
In the second case, we encounter Charybdis: we do not know all the ingredients and structure of causal relations in the new group, therefore, we can only treat it with the information acquired in the study of another group thus resorting to induction, the failure of which was discussed above. So Charybdis entails P-Kong.
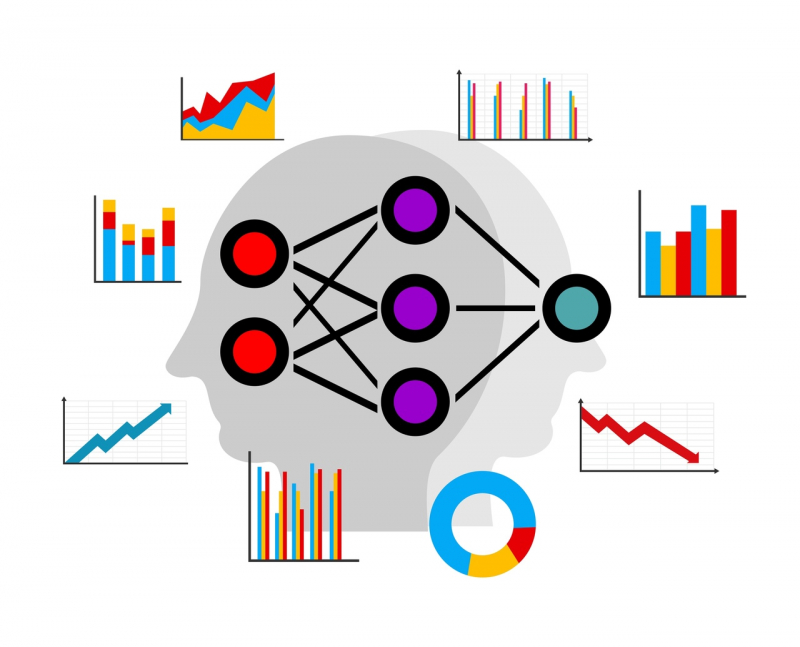
How to fight monsters? Causal inference as a solution
How do we solve all these problems? The lecturers suggest abandoning the simple policy of transferring successful decisions from one group to another. Instead, it is necessary to study the composition of significant factors and causal structures of each group where a particular solution was effective or ineffective. Based on such a study, it is possible to identify a more general structure of causality and the interaction of various factors. In this case, we resort to abductive inference.
With abduction, we do not simply copy and paste a solution that once proved successful, but try to form a more complete picture of causal relationships when we try to find an effective policy. By expanding the model, constantly rebuilding it and adding new data, we can make more accurate predictions and, most importantly, be more flexible with our solution.
The lecturers refer us to the models of causal relations that are currently used in AI in the creation of Bayesian networks.
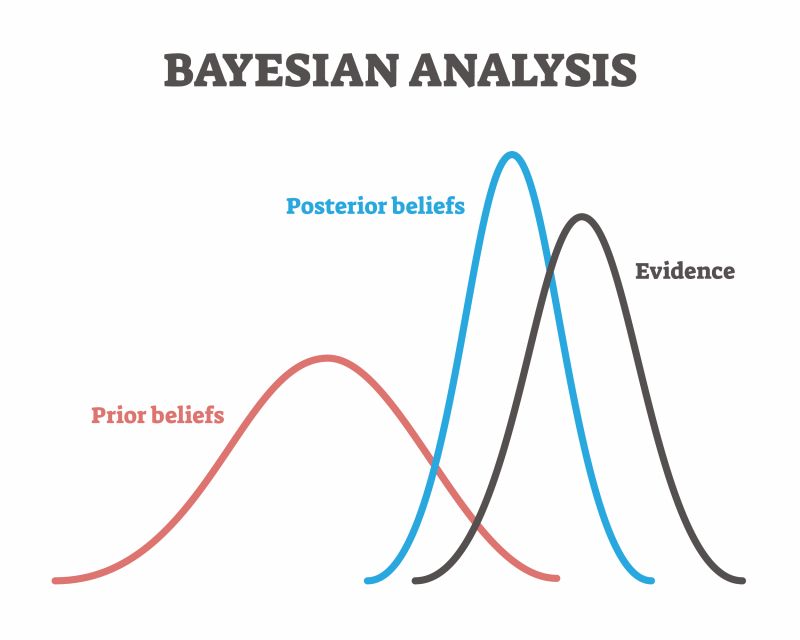
To be more specific, here are the steps to follow when constructing a causal model and the associated abductive inference:
-
We recreate the general model of causal relations that best explain all the previously acquired data (abduction-based).
-
We test and improve the model using new data and proofs (abduction-based).
-
We do a comprehensive research of all the factors involved in the situation we’re working with – and that we need to find a solution for.
-
We use all of the above to create a predictive model that will help us understand whether we need to modify our solution and how to do it. The more factors we can include, the more accurate our predictions will be.
The future of decision-making theory
The future of science lies in rejecting the traditional evidence-based methods that work exclusively on establishing causal relationships based on research data and accurately established facts.
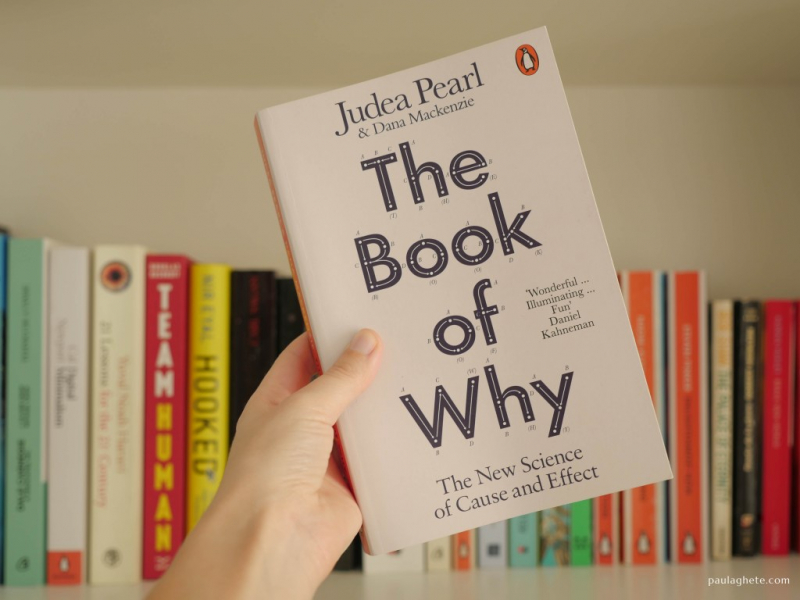
In The Book of Why, Judea Pearl, the creator of the mathematical apparatus of Bayesian networks, as well as the mathematical and algorithmic base of probabilistic inference, says that we should move away from the simple statements of facts and statistics when we construct our theories, but think more about why this or that event occurred, what is behind this or that phenomenon.
We must consider the whole situation, the whole set of factors, and not dwell on individual facts. He refers to the phenomenon of causality revolution in AI: to become closer to humans, machines must learn to operate the category of causality.
It is very likely that in the future, AI and machine learning methods will be actively used in political decision-making, which will allow us to analyze much more data – in its entirety, make more accurate predictions, conduct experiments, and test hypotheses before applying a particular solution.