Instagram me vs Real me
Social networks give us an opportunity to share personal updates with lots of people at once. At first, it seemed like a great idea – you can publish something once and receive feedback from everyone, rather than retell the same story to everyone. But as it turned out, not many people are very interested in your thoughts and insights. We started to believe that people like images more than they like ourselves in particular.
Modern people are much less excited about presenting their true selves to the public. All posts get filtered and social media representation differs from reality: that’s why “me on Instagram vs me in real life” memes appeared. Only tips of an iceberg are published on social networks these days.
Such “insincerity” is challenging for researchers. For example, during the last presidential election in the US, no algorithm predicted that Donald Trump would win. The thing is, people didn’t want to openly support him on social media, so algorithms only detected potential votes for Hillary Clinton. People adapt to certain expectations, so we end up with results that don’t correspond with reality. If we would take a look at the analysis of such data, we would see that it’s all about positive, socially acceptable images that distorts prediction results.
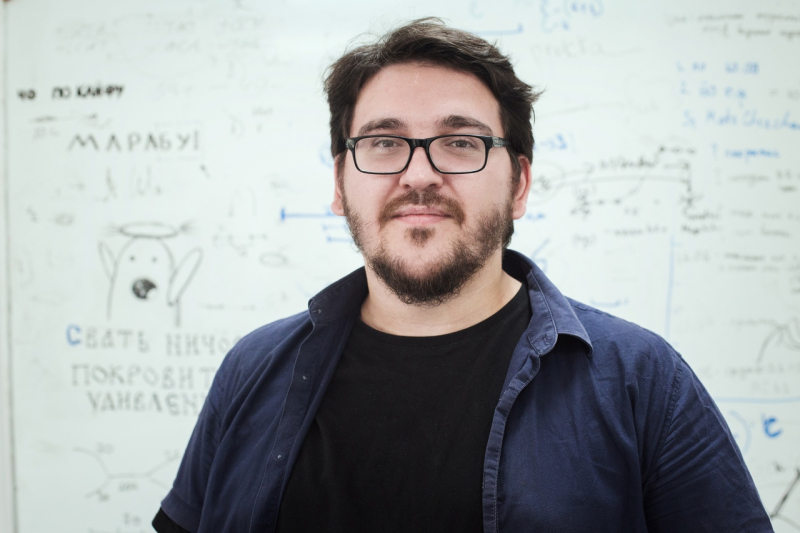
Social media instead of CV
Many companies ask potential employees to share their social media profiles. On one hand, it makes sense, as each employer would like to know more about personal and professional qualities of an applicant.
On the other hand, it’s reckless to demand this. Firstly, not everyone has social media profiles. Of course, few people don’t use messengers or social networks these days, as it’s hard to communicate without them in the modern world. Young people aged 20-25 without accounts on social media are thought of as something weird. However, such people exist, and we can’t presume anything about them just because they don’t use social media.
Secondly, the more widespread this requirement becomes, the more people will try to fit in by editing their content even more. We will be at risk of ending up with LinkedIn-lookalike profiles. People will only publish what potential employers expect from them. Or they will create several profiles, like teenagers who have separate (and very different) pages for parents and friends. Plus, bloggers will start to recommend what to publish in order to get hired.
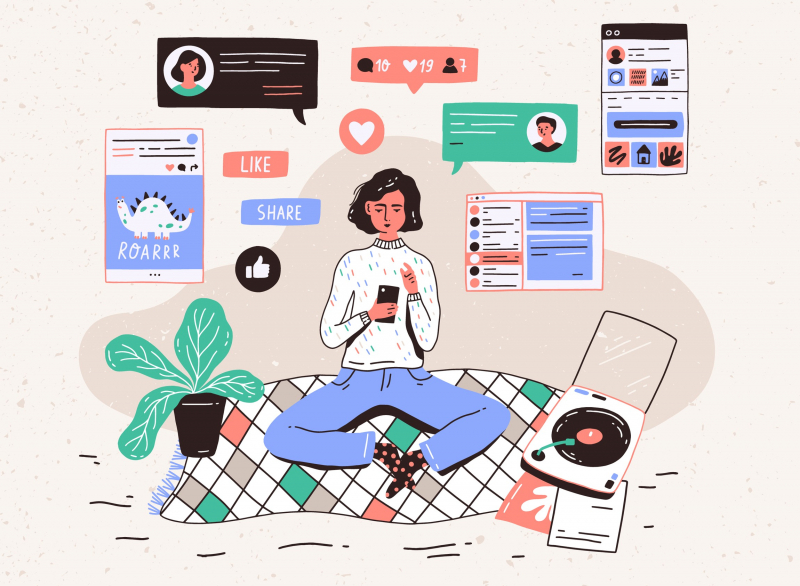
Looking for hidden info
One of the projects by our lab has to do with algorithms that use data from social networks to determine what isn’t in plain sight. In particular, we can determine psychological characteristics, relationship status, and income of users. It’s like Sherlock Holmes making conclusions based on certain details. His logical sequences were about predictions and sometimes he missed: for example, tobacco stains on someone’s clothes might’ve appeared due to someone else smoking near them – it doesn't necessarily mean that the person with stains was a smoker. That’s pretty much what we’re doing as well – a probabilistic prediction. The more data we have, the more precise it is.
It’s a long-term project that lies in the foundation of SoMin.AI, a startup by Aleksandr Farseev. It’s especially relevant for businesses. We live in the age when processes of production and transportation are well-established, so targeting becomes the key aspect. That’s what we help companies with. For example, we can predict quite precisely how much a person earns and then conclude whether they should see a Ford ad or a Lamborghini ad. Our algorithms are also used by employers. With their help, they can understand what kind of a person an applicant is even before the interview.
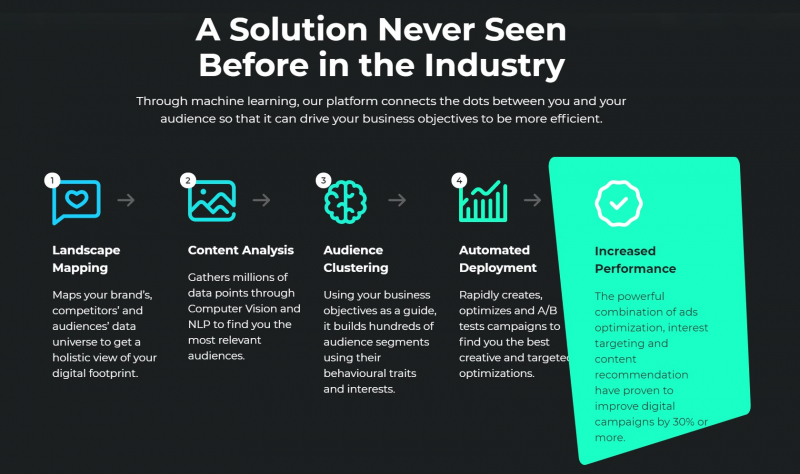
AI ethics
AI developers are often asked if we’re fine with the fact that our algorithms are used for this or for that purpose. In the professional community in general, the AI ethics are also discussed, but actually, it’s more about algorithms of decision-making. For example, no algorithm would predict whether you should hire someone or not. Our algorithms are just tools for the measurement of certain characteristics – the rest lies on our customers.
Each person has their own idea on what’s ethical. For example, some people don’t like it when our algorithms are used for advertising. They think that ads are one of the tools for manipulation and deception. I don’t think so. Targeting not only helps increase revenue of companies, it's also helpful for consumers – they see only relevant ads for products they can afford.
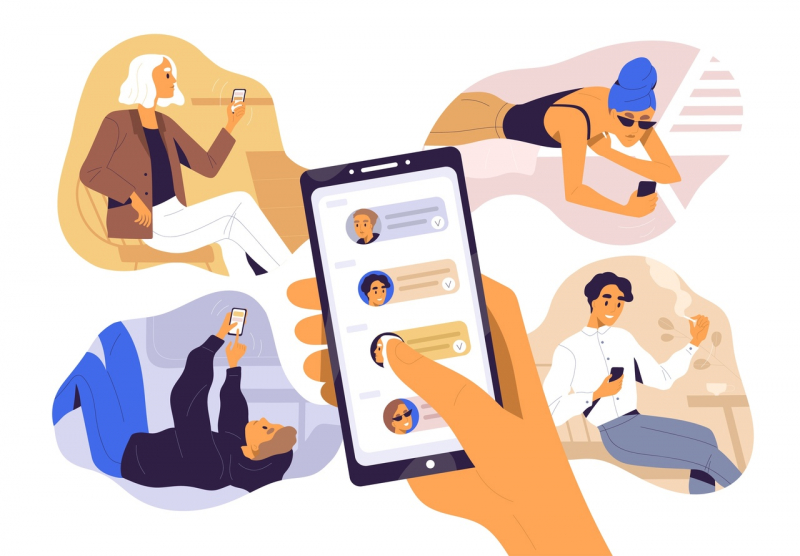
Here’s another example. In our society, even HR managers without gender prejudices are supposed to consider controversial criteria when hiring. Due to Russian labour laws and cultural norms, employers are more reluctant to hire women, as it’s assumed that they are more likely to take maternity leave and stay with children. So, from the employer’s point of view, it’s easier to hire a man – he will work a lot and won’t take maternity leave.
Of course, employers can determine the sex of an applicant without algorithms, but let’s say they decide to use our tool for this purpose. In this case, the problem lies not in the company or algorithms, but in our cultural norms and stereotypes. If we are to change something, it should be outdated beliefs and decision-making rooted in our society.
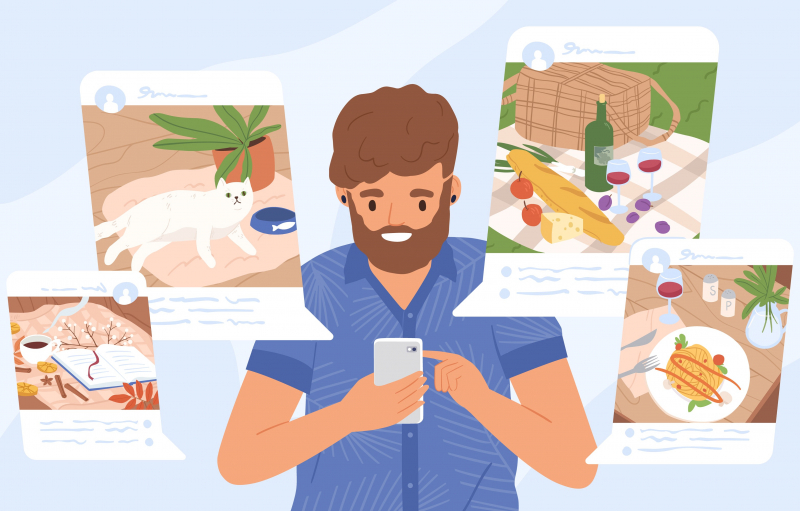
Challenges
In addition to the fact that not all user information is true, there are other challenges. Firstly, data is presented differently in each source. All sources require an algorithm created for them specifically. The more data we integrate, the more complex our tools must be. Moreover, each algorithm must be designed, trained, developed, and tested. You can't just take it off the shelf. Secondly, information necessary for training isn’t always open. You might need to do extra work. For example, to predict whether a person will support a particular politician or buy a particular brand of clothing, it’s not enough to simply collect available data. It’s also crucial to come up with a specific characteristic which will allow us to predict for whom they will vote or what they will buy, and only then train the algorithms.
Thirdly, data mining in social networks is tricky. Informational design and policies regarding what can and cannot be downloaded often change. In addition, the language in which people communicate is constantly changing: there’s slang, specialized vocabulary, and different symbols. Both social networks and ways of interaction change. For example, when VK just appeared, it seemed that the behavior of people on this site would pretty much always stay the same, and that you can come up with models to use in the future. But everything turned out to be different. Previously, people on VK often posted stuff on each other’s timelines, but they don’t anymore. People who discuss politics at some point left VK and joined Facebook. At one time, it seemed that Twitter would die, but it’s now more alive than ever, and, for example, that’s where the professional machine learning community communicates.
And finally, messengers like Telegram appear. Gradually, they acquire the properties of social networks, creating competition for them. Data from Telegram is harder to read, because it’s secured and you can’t see who follows this or that channel. In this sense, our expectations of an inexhaustible flow of open information that can be easily analyzed aren’t justified.
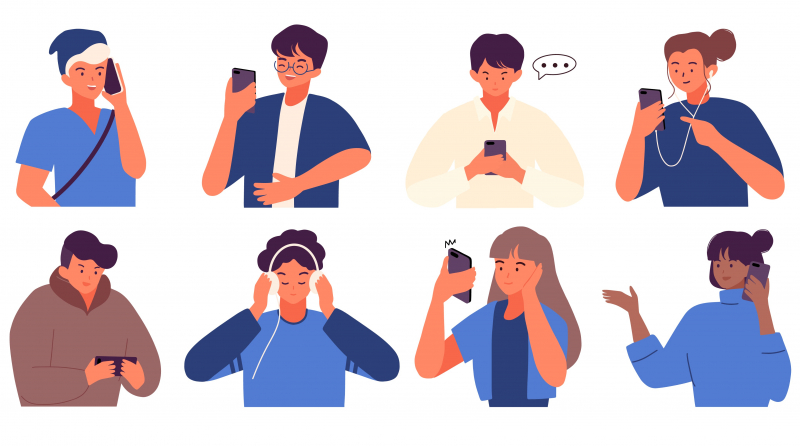
Developing an efficient algorithm
Social networks offer lots of opportunities for artificial intelligence developers. Based on the analysis of everything that the users of VK, Facebook and Instagram publish on their pages, both recommendation systems and algorithms are being developed to reveal additional, hidden information: for example, psychological characteristics, income, and relationship status.
In order to create efficient tools that would be useful both for users and businesses, developers need to keep an eye on all the changes and be ready to adapt to them. Human behavior and language change over time, and so do social networks.
Speaking of the ethical side of AI, it’s important to remember that algorithms are just tools that help reveal certain information. They are neither bad nor good. The responsibility for their application lies entirely with those who use them. Accordingly, the question isn’t about how to make this or that tool more ethical, but how to make people and developers themselves think more ethically. But even before that, it would be nice to determine what we understand by the word “ethical”.