The functioning of existing AI algorithms is provided by conventional electronic devices, whose power is already comparable to that of the human brain. However, their architecture limits the full potential of neural networks. Current platforms for neural networks function based on the principles of the von Neumann architecture, where information is stored and processed by different units – this largely limits the speed of neural networks. Moreover, the Boolean logic used by such networks, too, differs significantly from the algorithms used by biological neural networks.
One possible solution to this contradiction is the creation of devices that follow the principles of neural networks in the human brain. The company Intel were able to make a significant step in this direction: their Loihi and Loihi-2 chips work similarly to biological neurons. This means that the neural networks based on these devices learn more quickly and achieve higher energy efficiency and plasticity. What still remains to be established is the optimal material for platforms at the core of neural networks. Parts for such devices were suggested by ITMO University researchers. Their MOF (metal-organic framework)-based systems are activated by a laser pulse and imitate the behavior of neurons: when the frequency of a stimulus changes from low to high, the systems respectively change their response from active reaction (potentiation) to passive (depression).
The suggested crystals are MOFs, a class of materials discovered in the 2000s. They are made from building block-like organic molecules, with metal ions as their connective links. Usually, MOFs are produced from a chemical solution of the two chosen compounds.
In their research, ITMO scientists used crystals based on copper and trimethylic acid, which served as the organic component. The components of such materials are easily subject to organic decomposition, making them harmless to the environment. Another advantage is their relatively low production cost. Unlike the rare and difficult-to-extract silicon, which is currently used in all electronics, these crystals can be made in a test tube in any laboratory, yielding results within an hour.
“If a laser is directed at a crystal with a one-second pause, the intensity of its reaction to the light gradually decreases. If the pauses are increased to two seconds, the intensity increases. This behavior of the crystals is similar to the reactions neurons display to neurotransmitters (biologically active substances that play a crucial role in transmitting information from one neuron to another). A vivid example is the effect of serotonin, or the “happiness hormone,” on our brain. The more frequently we eat chocolate, the less pleasure we derive from it; conversely, the less often we indulge, the more intense our emotions are. This dependency of the crystals’ reaction on the frequency of stimulation allows them to be used for neuromorphic computing, which involves logical operations that are analogous to those performed in the brain,” says one of the paper’s authors Semyon Bachinin, a PhD student and junior researcher at ITMO’s Faculty of Physics.
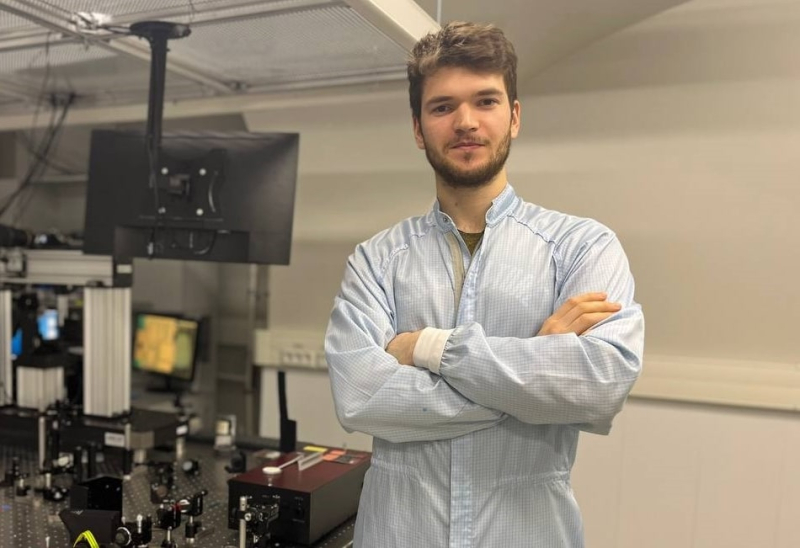
Semyon Bachinin with the installation used to study the crystals. Photo courtesy of the researcher
With such crystals, it’s possible to encode, transmit, and store information faster and more efficiently. In order to do this, the crystals have to be subjected not only to a laser, but also to electric pulses. A combination of electric and light pulses of different amplitudes can switch the crystal between several electric states, which creates its capacity to work with information.
All computational operations are made within a single crystal rather than across different units, as is the case in traditional electronic devices. This results in reduced time and energy required to perform all computations. Scientists refer to this concept of storing, transmitting, and encoding information on a single element as in-memory computing. This technology is currently being actively developed worldwide. For example, tech giants like Google are eager to implement such solutions to accelerate data processing. The crystals created by ITMO scientists could also facilitate the transition to similar technologies, allowing for environmentally friendly production and the use of Russian-made components.
During their experiments, the researchers compared the speed and number of training cycles of neural networks specializing on handwritten text recognition that worked on a conventional device and on the developed crystals. Tests show that the neural network based on the crystal learned to recognize handwritten text with a 100% accuracy quicker and more efficiently, as well as performed over 50 recognition cycles.
This research project was conducted within the program Priority 2030.