What issues is your scientific group trying to solve right now?
In short, at the core of our research is the problem of heart arrhythmia: its causes and possible treatment methods.
Atrial fibrillation is known as the most common irregular heartbeat. There is evidence that this disease is associated with an increased risk of stroke. On the one hand, it’s easily diagnosed, but on the other, it remains a serious problem. Why so?
Atrial fibrillation is indeed pretty easy to diagnose. By simply looking at your ECG results, any physician will tell you if you have it or not. But many people don’t do check-ups, as they don’t feel any symptoms.
However, the main problem with atrial fibrillation is that we don’t really know how to treat it. There are some treatment methods but they don’t work for everyone. But even when they do, they only work for a couple of years. Most of them are also quite expensive. Our aim is to offer people effective and affordable treatment.
During your lecture at ITMO University, you mentioned that all heart modeling approaches are based on fundamental research. How do you combine computer technologies and fundamental science in your work?
The first thing to think about is that our heart is composed of cells. But if we only examine one cell, we’ll never understand the mechanism of arrhythmia since it doesn’t develop at a single-cell level, but at an organ level, while every organ is composed of a myriad of cells.
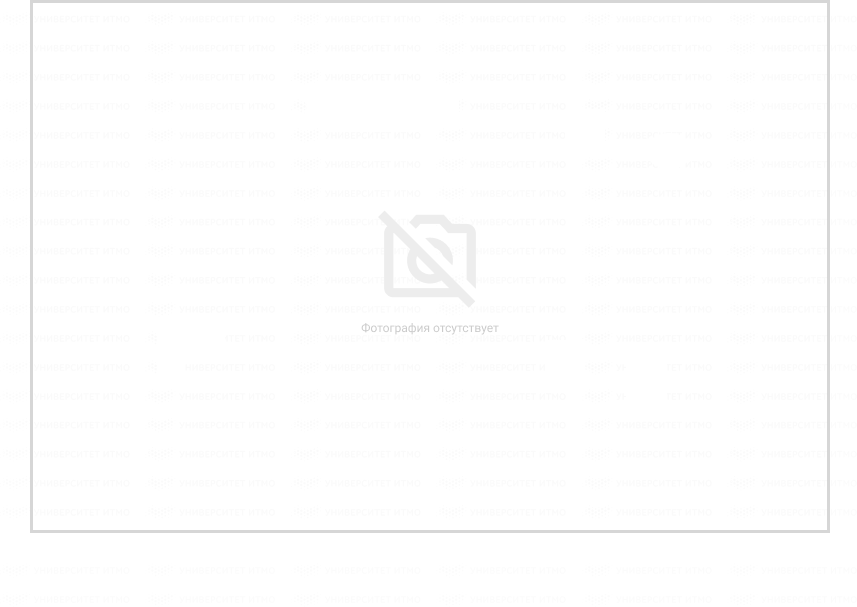
For example, genetic scientists can examine a group of people who most often die from arrhythmia. But what they do is they search for a mutation in certain genes, which doesn’t explain why people die from this disease. To solve this problem, we have to approach this question from a broader perspective and study not a single cell but the whole heart. So after we find a correlation between certain mutations and arrhythmia, we can think of the ways these mutations can be cured. This is one of the examples of how fundamental science can be used to solve real-life tasks.
There is also another approach, aimed at offering each patient individual treatment using computer technologies. Scientists hope that in the future, every patient will not only have their own electrocardiogram but also their own heart model, which will help physicians examine each case in more detail and prescribe the best treatment.
Having majored in physics, how did you come to start applying your knowledge and skills in the field of biomedicine?
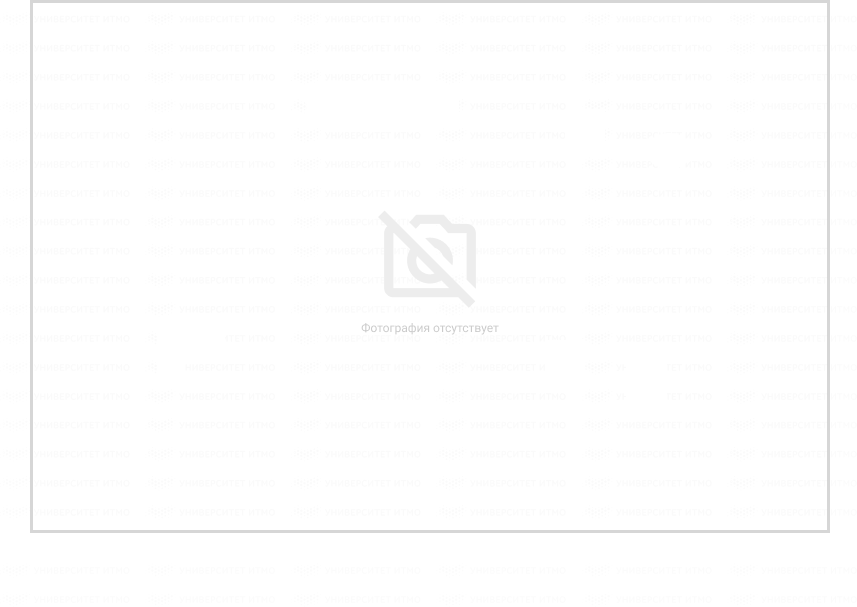
Though I studied at the Moscow Institute of Physics and Technology, I’ve always been interested in biology. From my very first year at the university, I knew that I wanted to do biophysics. But back then, theoretical physics was very popular at MIPT; I applied and somehow got accepted. After a while, I realized that theoretical physics was not exactly my thing, as it’s too abstract. Then I went to Pushchino, where Prof. Krinsky used to work, and he offered me a job.
I’ve been studying nonlinear waves my whole life, and I can say that this sphere has changed dramatically over the last few years. When it all began, we were the first in the world to work in this area, while nowadays nonlinear waves are researched in almost every laboratory where they study the heart.
As for spiral waves, it all started as an academic project and evolved into some serious practical applications. Special devices that are able to detect spiral waves in the heart are already being produced. What is remarkable is that the research conducted in the Soviet Union contributed greatly to the progress in this field in Western countries.
What specialists are needed in this sphere?
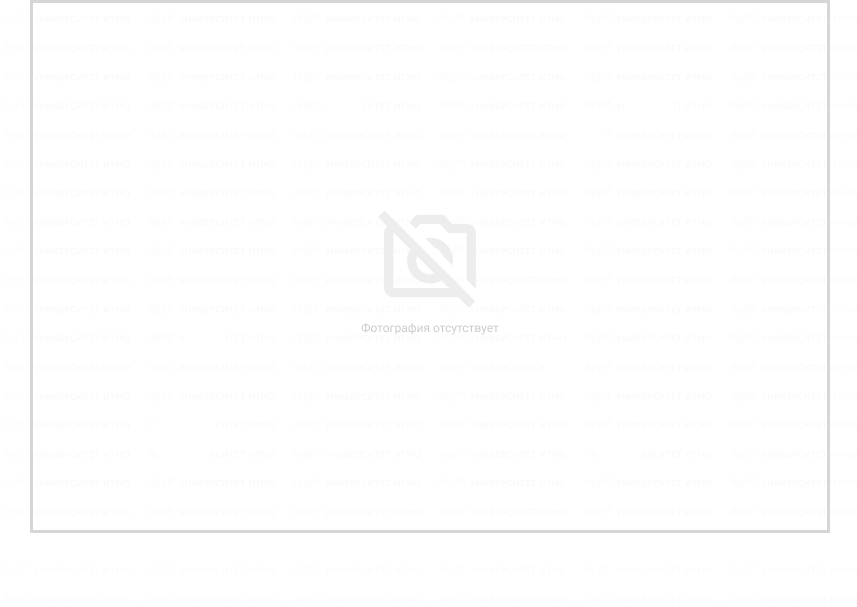
One of the modern trends in science is multidisciplinarity, however, it’s still not easy to find a specialist in several fields. As for the background, we welcome specialists in different fields, from bioengineering to mathematics and physics.
What about the field of computer science?
Computer science is now taking the scientific center-stage by storm: computer methods are becoming to be actively used in our research field, too, and this is why we’re very interested in cooperating with ITMO University, inter alia. Before the computer science revolution, we were faced with problems which were seemingly unsolvable, but can now be tackled with the help of artificial intelligence technologies. This is a whole new ground with a myriad of tasks we can explore.
I came to ITMO to lay the foundation for future cooperation and discuss the possibility of creating a research group as your scientists also work on cardiology issues drawing on the methods of computer science. We’ve already established links with the Almazov National Medical Research Center, which is considered one of the best medical centers in Russia (ITMO University also collaborates with the Almazov Center which is part of ITMO’s National Center for Cognitive Technologies – Ed.).
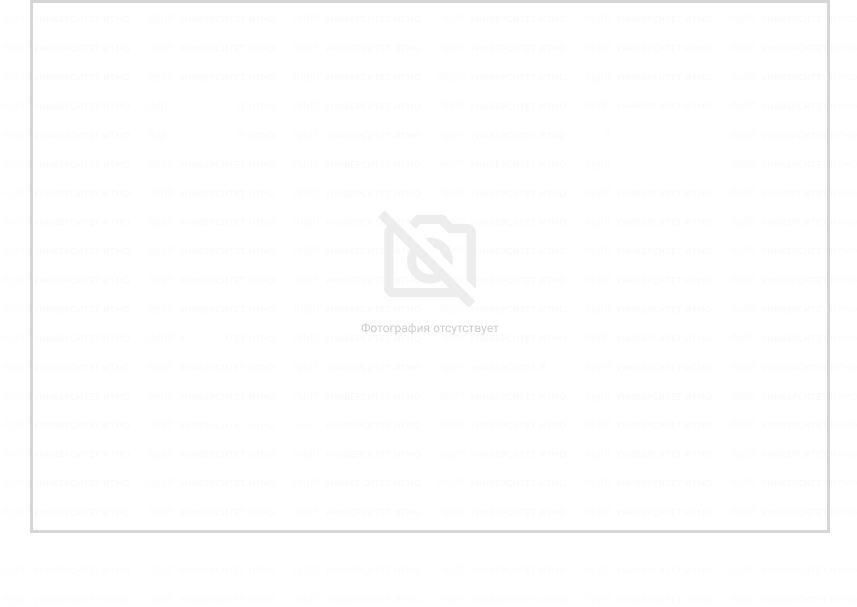
There we work with Prof. Dmitry Lebedev, an acclaimed Russian arrhythmologist who heads an extremely prolific research group comprised of talented young scientists. Our partners from the Almazov Center are very interested in innovations, and this is an important factor in our cooperation.
How difficult is building this practitioner-scientist interaction?
Establishing a good working relationship with medical practitioners is a unique achievement for us scientists. This is a very difficult task indeed: doctors are extremely busy people; moreover, this profession has lots of limitations regarding the storage and processing of personal data. I’ve traveled all around the world trying to get doctors on board. The most frequent answer I got was ‘no’: practitioners aren’t that keen on participating in scientific research, they just treat patients and get paid for that. That’s why building an effective interaction with doctors is a rewarding but hard work; as a general rule, these relationships take years to create.
Continuing the topic of interacting with computer science specialists: exactly which problems can they help you with?
I believe that at an early stage, we’re not requiring anything extraordinary. The field of computer science has developed a set of effective research methods, and we just need to have them applied to our research objectives. We have already identified specific tasks that can be solved in this way. It’s widely known that machine learning allows us to deal with tasks that require facial recognition. Our problems are very similar in their core. Take the case of measuring cardiac electrical activity. The best way of doing that will be to place a so-called basket catheter inside the heart; this device looks a bit like a torch for children. After we insert it, it opens up to give us 64 electrodes that help to record the position of a wave.
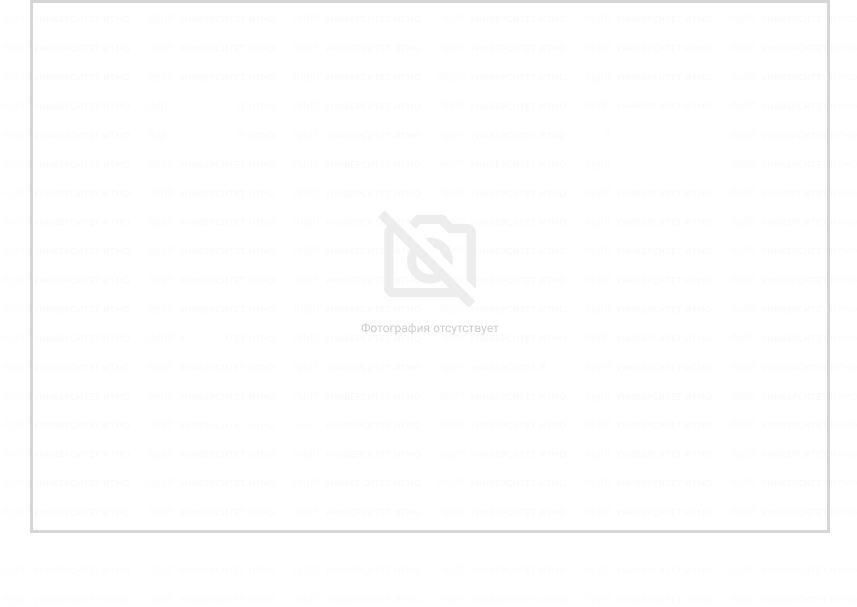
Specialists are faced with the task of how to use these recordings for identifying the sections of the heart that are responsible for developing cardiac arrhythmias and eliminate those through the procedure of ablation (or local cauterization). You can decide on whether or not this is a difficult task yourself by downloading an app created by my colleagues from King’s College London.
This app allows you to see the procedure via an ultramodern smartphone with a display that consists of thousands of pixels, which in this case represent electrodes. But what to do if you only have 64 electrodes at your disposal? You’re forced to work with images of a very low resolution. That’s why our first task for computer science specialists would be to improve the quality of this image and only then move to the process it represents. This is one of those classic problems we need to be solved.
Another major issue in our field is the massive amount of big data. Nowadays, many patients make use of technologies that produce a detailed electrocardiogram tracing of their heart. These solutions encompass hundreds of thousands, and maybe even millions, of people with cardiac illnesses. These data can and need to be analyzed. I’ve been granted a visit to the Spanish National Centre for Cardiovascular Research recently, and its specialists introduced me to the results of their work with patients who suffer from atrial fibrillation. They’d gathered an insane amount of data and used it to forecast how the frequency of this kind of arrhythmia would change over time. This is important as it enables us to estimate what will happen to a specific patient in three or five years and when they need to be treated.
Do you also work with user data from mobile apps and trackers?
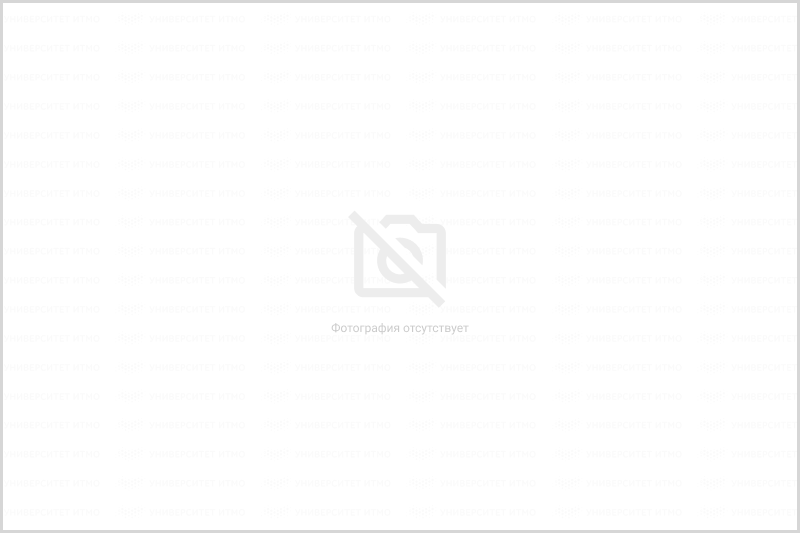
Because of how hard it is to obtain, we don’t, as of yet. The companies producing such devices are reluctant to share this data. But there are special international consortiums which we can approach to obtain this information. It would be great if these data banks existed in Russia.
You’ve mentioned that one of the global goals that the researchers in your field dream of achieving is to make it possible for patients to have true-to-form models of their own heart, which will give them the opportunity to receive personalized treatments. What developments in this direction can we expect in the near future?
Personalized therapy is a question of our present, not our future. There have already been publications in Nature Communications (reference: Arrhythmia risk stratification of patients after myocardial infarction using personalized heart models, Nature Communications volume 7, Article number: 11437, 2016) and Nature Biomedical Engineering (reference: Personalized virtual-heart technology for guiding the ablation of infarct-related ventricular tachycardia, Nature Biomedical Engineering volume 2, pages 732–740, 2018), the authors of which worked with specific patients to develop personalized models that were then used to come up with a set of particular recommendations for an individual therapy program. This is all very real, but there are different levels of what we can achieve. The highest one, the one we should reach for, implies examining a person’s heart, building an individual model, and forecasting a suitable treatment, so that a surgeon can perform a case-based operation trusting these findings a 100%.
But there are lots of interim levels leading to this global goal: even now, we have all what it takes to create specialized software that would advise doctors on the appropriate treatment, to help them in their decision-making. These technologies are already available, and we can use them to speed up surgeries, at the very least. But I expect that in the future, when this technique is more established, people will trust it more and it will become an integral part of the medical practice.
Alexander Panfilov is a professor at Ghent University (Belgium) and at the Institute of Cardiology of the Leiden University Medical Center (the Netherlands). He is also professor emeritus of the University of Dundee in Scotland, where he teaches Maths. Dr. Alexander Panfilov’s research interests include computer modeling of biophysical processes, studying mechanisms behind the phenomenon of sudden cardiac death, and developing treatments for heart arrhythmias. The scientist is the author of more than 190 research publications in the leading scientific journals, an expert at the Wellcome Trust (UK), a member of the Physiological Society (UK) and Heart Rhythm Society (USA), and an editor of three scientific periodicals.