Bioinformatics Bootcamp was a four-day intensive training in which teams studied data analysis tools and techniques under the supervision of mentors, participated in expert-led workshops, and worked on projects in genomics and metagenomics. They sought new forms of bacteria in Russia's ecosystem, investigated the effect of microorganisms on human health, developed virus detectors, evaluated the influence of mutations on gene structure, and addressed a number of other problems.
The hackathon was attended by 120 students and graduates (24 teams) from St. Petersburg, Moscow, Kazan, Voronezh, Minsk, Rostov-on-Don, and other cities. Among the event’s partners were Genotek, Zoogen, and Serbalab.
All participants who presented their projects will be accepted into the Applied Genomics Master’s program following an interview with the head of the program. They will also be able to work on real-world projects by the Applied Genomics Laboratory, as well as the program’s academic and industrial partners.
The jury board picked three winners on June 27. The evaluations were based on four major criteria: research design, findings, quality of presentation, and anticipated benefits.
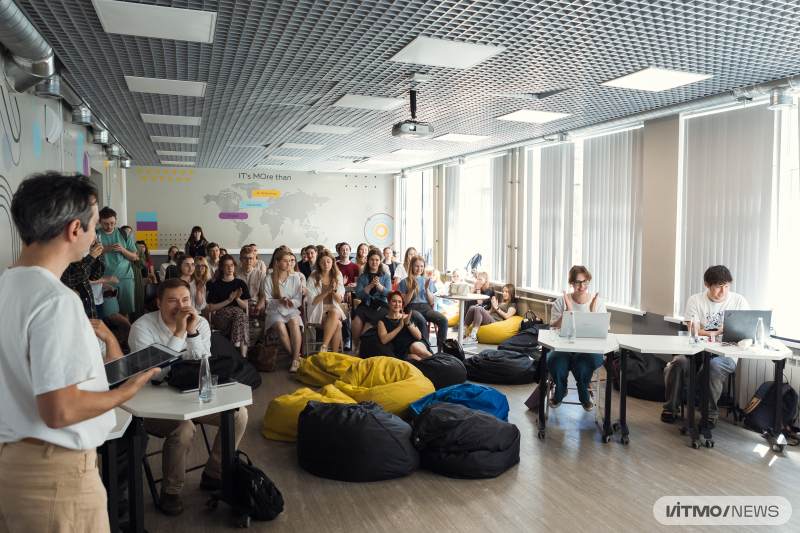
The final pitch session. Photo by Dmitry Grigoryev / ITMO.NEWS
Projects
The second place was shared by two teams with the same score – Krons and My Little Protein.
A study of gut microbiota composition. For their project, the members of the Krons team examined samples of the large intestine, which has the highest bacterial load in the human body. The samples were collated from both healthy people and those suffering from gastrointestinal inflammations, specifically irritable bowel syndrome, Crohn's disease, ulcerative colitis, and colorectal cancer.
The study found that healthy people and patients with inflammatory bowel diseases shared 114 taxonomic ranks of bacteria; however, each condition has around 3-7% of its unique ranks. According to the team’s leader, Valeria Koss, the study's findings might be useful for illness diagnosis and therapy based on a targeted effect, such as diet, medications, or fecal transplants.
Search for a regeneration gene. The team My Little Protein studied the genome of the spiny mouse, which is most recognized for its remarkable regeneration ability: it can regrow its skin or tail without scars.
The researchers examined the mouse genome for proteins that may be connected with their enhanced regeneration abilities. As a result, two proteins were identified as having elevated expression during healing – the anti-inflammatory protein interleukin 10 (IL-10) and the matrix remodeling proteins Mmp2 and Mmp9.
“It’d be interesting to compare our results with those acquired by analyzing proteins of other regenerating organisms. In the future, our findings can be used to create wound healing medications,” says Tatiana Sizova, a member of the team.
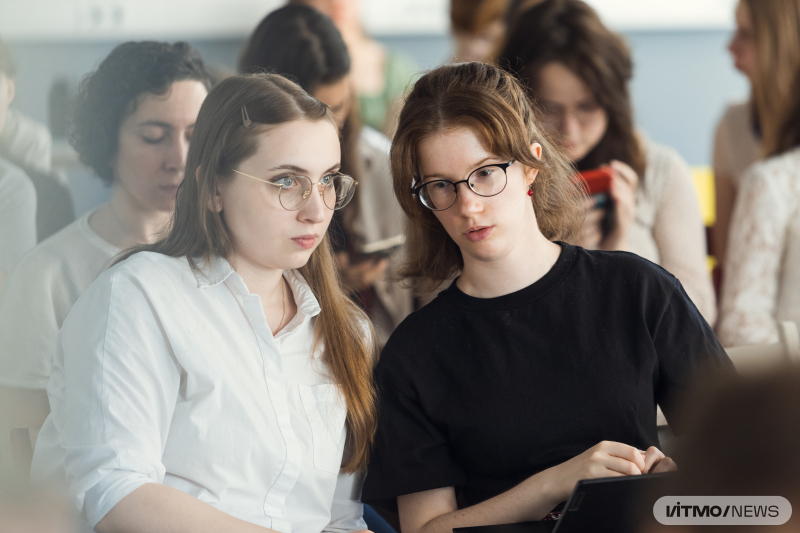
A hackathon participant and Tatiana Sizova (left). Photo by Dmitry Grigoryev / ITMO.NEWS
An ML model for gastritis diagnostics. The first place was taken by the team named Slavные парни (Slavnye Parni, or Good Guys). The team studied databases of intestinal microbiota samples from patients from four Russian regions: central, southern, south-western, and Siberia. The data contained the compositions of varied bacteria in colon microbiota samples. The young scientists looked for a correlation between a person’s microbiota and their place of residence, as well as developed software that could diagnose gastritis based on gut microbiota.
The study found no relations between a person’s place of residence and their intestinal microbiota. The model Gastritis.ai demonstrated an average accuracy of 97%. With its help, the team discovered the bacteria that can become indicators of gastritis: Faecalibacterium prausnitzii, Bacteroides uniformis, and Ruminococcus bromii.
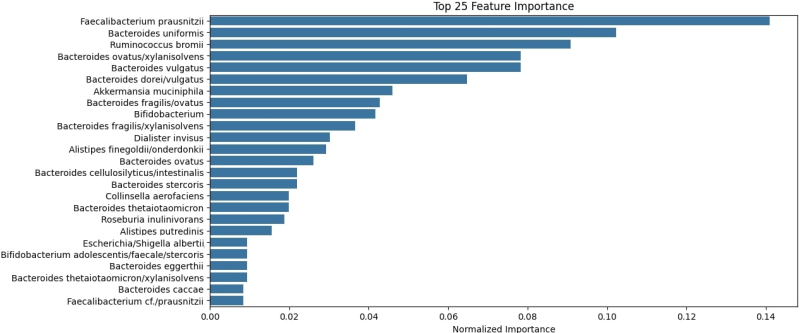
The average accuracy of the Gastritis.ai model. Illustration by the organizers
“Aside from creating a diagnostic model, we also managed to find potential indicators of gastritis. Although Helicobacter pylori is the most prevalent cause of the disease, we were able to identify the condition based on a microbiota sample that did not contain any gastritis-inducing bacteria and thus highlighted the ones that should be paid attention to when making a diagnosis. A change in their number may indicate the development of the disease. Our study may be helpful in gastritis diagnosis,” explained Evgeniy Brilenkov.