Even though nowadays almost every smartphone is equipped with speech recognition technology, some fields associated with supra-verbal characteristics still pose a challenge to researchers. In doing so, such technologies can greatly improve the lives of some population groups. The recognition and analysis of emotion in the speech of the elderly and children remains an insufficiently studied and complex field of research that could potentially solve important and essential tasks related to the life and health of these groups. Speech technologies help create voice assistants to facilitate the life of the elderly, as well as devices for monitoring their psychophysical state. In the future, such devices will be able to analyze older people’s voices to determine if everything is in order with their mental and, possibly, physical health. One of the algorithms that could make this possible was proposed by ITMO University PhD students and staff members of the International Research Laboratory Multimodal Biometric and Speech Systems Oxana Verkholyak and Dmitrii Fedotov as part of an international research group.
Why should older people's speech be analyzed differently?
The first speech recognition device that could understand spoken numbers appeared in 1952. The development of this technology has made it possible to analyze not only the content of speech but also emotions, inflection, accent, psychophysical state, and other accompanying parameters. The science of paralinguistics studies the nonverbal characteristics of speech and its main focus is not on the content of speech but on the voice, which helps figure out the feelings of a speaker. Computational paralinguistic analysis is considered one of the faster-evolving fields in speech and language processing but it is still not universal.
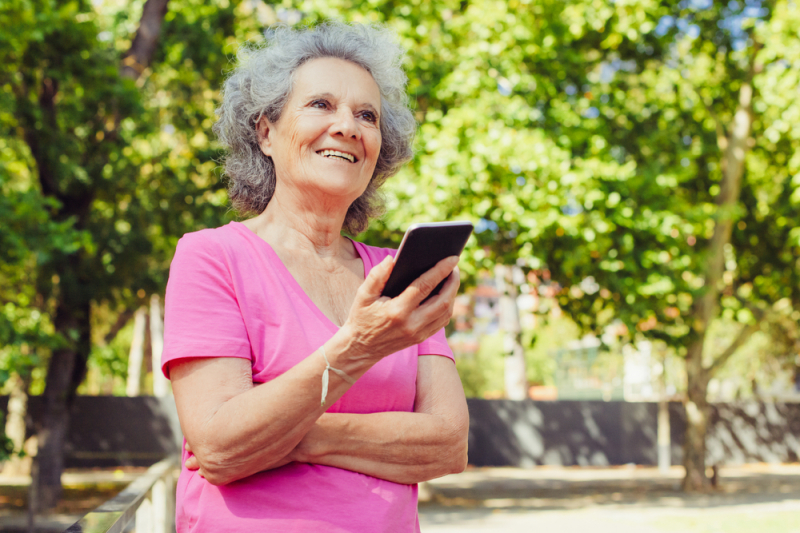
The lack of research on the speech of older people is caused by the lack of databases – the main subject of paralinguistic analysis. The acoustic characteristics of older people’s speech differ significantly from that of other age groups: their speech is less intelligible and has a lower tempo. In general, some vocal characteristics show signs of age. It means that available datasets cannot be used to develop models for speech recognition and analysis. Moreover, existing systems are also optimized for the voice of an average adult and are less accurate in recognizing the voice of an older person. The emotionality of speech complicates the analysis even more.
Today, scientists want to create a system for automatic recognition of the emotions of this particular population group. ITMO University researchers, as part of an international research team, have proposed a dual model for analyzing the speech of the elderly, which works simultaneously with the acoustic characteristics of the speaker's voice and the linguistic characteristics of their speech. The study was carried out in collaboration with the SPIIRAS Institute within the framework of a project on the complex analysis of paralinguistic phenomena in speech with the support of the Russian Science Foundation.
Not only what we say but also how we speak is important
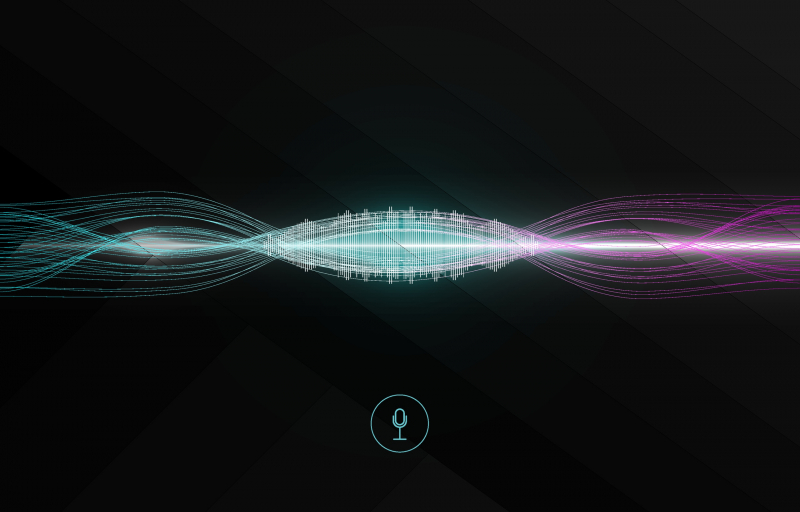
Paralinguistic analysis allows us to detect vocal characteristics that describe a speaker at a given moment. This is made possible by studying not what the speaker says, that is, the content of their speech, but how they say it. For example, the acoustic characteristics of speech are analyzed – these are the tone, timbre, strength, loudness, or intensity of the voice, the length of sounds, i.e. time spent on their pronunciation. These characteristics help to identify the parameter of speech “activation” – the excitation level of the speaker at the moment. The subject of acoustic analysis is usually audio files; in this case, recorded stories told by the elderly.
Also, the linguistic characteristics of speech – the very components of the utterance, that is, the words used – are important for paralinguistic analysis. Text units help to identify another parameter of speech and this is its “valence”, which shows how positive a person is. However, linguistic characteristics are more difficult to analyze and interpret.
“Words by themselves convey mainly the content of an utterance, and it is not always easy to extract signs of an emotional state from them. Usually, you can notice how emotions affect vocal characteristics, for example, the tone of the voice and its energy rise. But the interpretation of the emotional coloring of words requires the use of indirect criteria. That is the difficulty,” explains Oxana Verkholyak, a PhD student at ITMO University's Information Technologies and Programming Faculty.
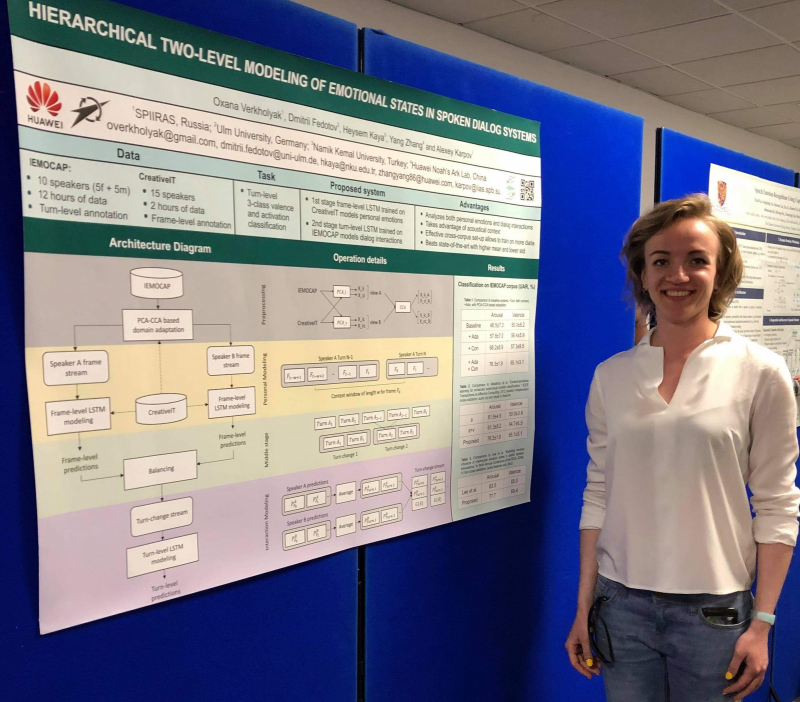
It is also easy to determine whether a person is calm or excited by their voice but it is difficult to gauge whether they are positive or negative. It turns out that acoustic models do a good job of recognizing the excitation level but they are worse at recognizing emotional valence. To create a universal model, the researchers used two modalities corresponding to the acoustic and linguistic characteristics of speech. They studied both activation and valence.
How does acoustic and linguistic speech analysis work?
Paralinguistic speech analysis is most often based on databases of recorded utterances and acoustic analysis uses audio files and linguistic analysis, namely texts. Each speech and vocal unit (tone, timbre, word, and so on) corresponds to a certain marker that reflects the speaker's attitude towards the content of their utterance. In speech analysis, it is usually one of six categories of emotional state: anger, sadness, disgust, happiness, surprise, and fear. Separately, the neutral state is distinguished as the absence of any other.
The model learning process takes place in two stages: feature extraction and classification. Broadly speaking, the modeling system remembers each speech and linguistic unit and the corresponding marker, and makes general conclusions based on this data. As a result, it correlates the units used by the person with the emotion that they experience during the speech. After training, the system is tested on new recordings. In acoustic analysis, the model used an activation scale from 0 to 10 as an output.
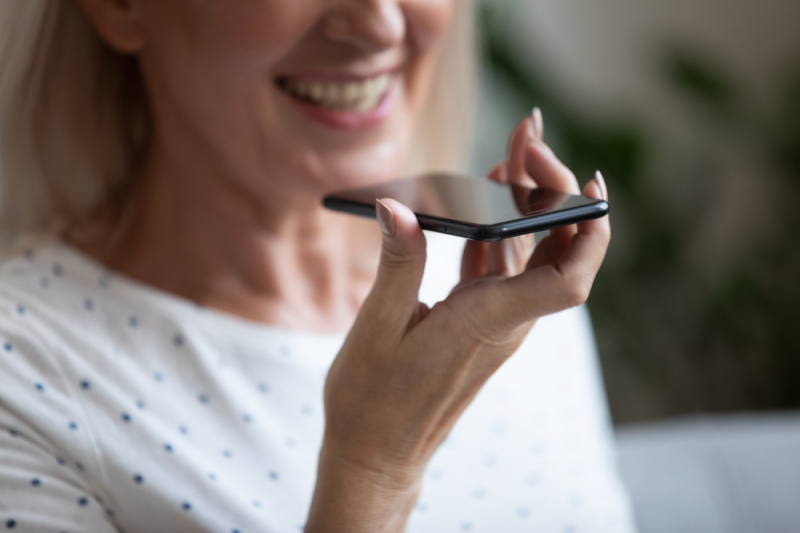
The researchers’ approach to the analysis of linguistic modality was to use tonal dictionaries. Tonal dictionaries assign a meaning of "positivity" or "negativity" to each word. The model determines the meaning of each individual word in an utterance and then creates the general picture, that is, determines the connotation of the utterance. The use of dictionaries also greatly improved the model’s performance with a small amount of data (speech samples of the elderly). In addition to vocabulary-sourced traits, the researchers used traits extracted from pre-trained neural networks. They were also collected from large text databases containing words and the context of their use. The scientists used several versions of the models that worked in an ensemble, which helped to increase the accuracy of the analysis.
It is possible to predict the emotion that the speaker is experiencing based on two indicators – activation and valence. For example, happiness has high activation and high valence, and anger has high activation and low valence. This means that a happy speaker is excited and positive, while an angry speaker is also excited but negative.
The study analysed German-language speech as it was carried out within the framework of an international competition in computational paralinguistics. The database of the speech and emotions of the elderly was provided by the organizers and compiled at the Ulm University. The sample consisted of 87 people aged 60-95. The study used two negative and one positive story. After each story, the mood of the participants was assessed according to the parameters of valence and activation on a scale from 0 to 10. The final database included audio recordings and transcripts of their speech.
What other research is already there?
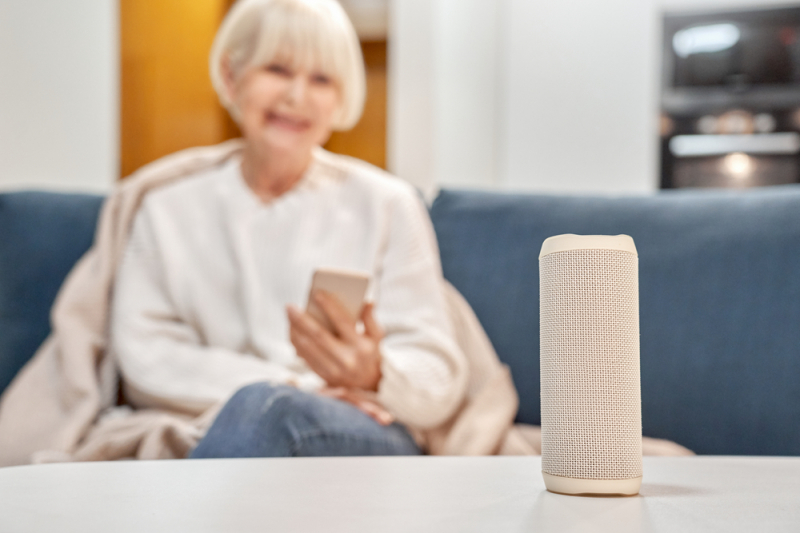
The elderly are an under-studied but vulnerable age group when it comes to voice recognition and analysis. They especially need support whether they are at home or in a specialized institution. As the population ages, the burden on the health care system increases, as does the need to improve technologies for caring for the elderly. Unfortunately, even in a hospital or nursing home, they may be afraid to share everything they feel physically and emotionally, so as not to bother staff. Speech recognition and analysis technologies built into smart devices can make it easier to monitor the psycho-emotional state of the elderly and diagnose possible diseases. “One of the signs of psychosis, a condition characteristic of many mental disorders, in which the “connection” with the real world is lost, and a person starts to see, hear and feel what is not really there, is a disruption of the thought process. It, in turn, can be manifested in speech impairment,” writes the N + 1 magazine, citing a study of the speech of patients with schizophrenia.
Scientists from several countries are already working on systems for automatic speech analysis of older people. For example, American researchers have proposed an algorithm that can identify lonely elderly people based on the acoustic and linguistic parameters of speech, and scientists at the Massachusetts Institute of Technology have developed a model that can diagnose depression. Countries with actively aging populations are also designing digital technologies for helping older people. In Japan, speech databases are being created for the elderly to improve the accuracy of their speech recognition. Humanoid robots equipped with recognition technology are being developed for use in nursing homes. Interacting with them can not only enable older people to communicate but also preserve their cognitive abilities. Also, algorithms that recognize emotions and are used in any voice assistant can adapt to the psycho-emotional state of the interlocutor.
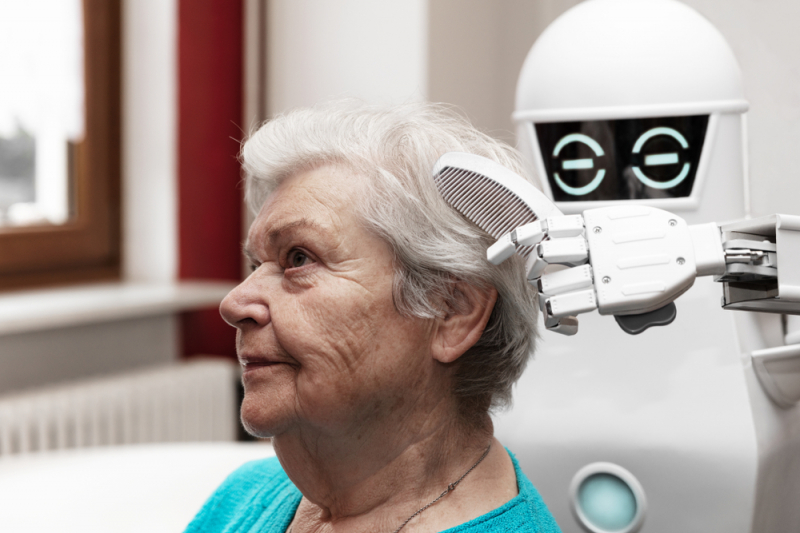
It's worth bearing in mind that the level of high technology adoption in Japan is likely to be higher than in Russia. Therefore, it is difficult to accurately predict how robotic assistants will be perceived in nursing homes and hospitals in our country. However, the biggest obstacle to the development of speech analysis technologies for older people remains the lack of relevant databases.
“It is the analysis of paralinguistic phenomena that can be used as a secondary system for speech recognition technologies. Emotional speech is recognized worse than normal speech due to changes in vocal characteristics. However, if the system already knows what emotional state a person is in, it can adapt to it and better understand the speaker,” Oxana explains the prospects for automatic recognition of the emotions of the elderly.
Multimodal emotion analysis systems can help expand the technology. For example, adding video recognition – the best source for valence recognition – will make it possible to read moods literally from a person's facial expressions and thus predict their emotional state. In general, these technologies for collecting emotional data will work best in combination. Digital assistants based on them will make life easier for older people. They will also be able to automatically monitor their mental and physical health and report any changes.