Choosing treatment and reorganizing the healthcare system
The lab’s specialists are engaged in predictive modeling for medical and healthcare purposes. Almazov National Medical Research Centre, one of the leading cardiology centers in Russia, is the main academic partner of the lab. The researchers work with a depersonalized copy of the patient database – all personal information is deleted and they can only access medical data.
According to Sergey Kovalchuk, there is a wide range of possible tasks for research: from choosing a strategy for treatment to organizing various processes in a medical institution or the entire healthcare system.
The lab’s staff members not only do research but also share their experience: in June, they organized the second workshop as part of the International Conference on Computational Science conference that was attended by participants from 32 organizations in ten countries, such as Poland, Australia, the US, Netherlands, and Germany. Overall, there were 27 presentations at the conference.
Together with Almazov National Medical Research Centre, the researchers presented a paper on modeling the development and treatment of diabetes. It’s a continuation of research presented at a workshop last year and chosen for a special issue of the Journal of Computational Science.
“We apply various tools of predictive modeling in order to detect and study what typically gets neglected in the healthcare system. We can do it at different scales. For example, we can optimize ambulance dispatching and routing, or optimize the patient flow in one hospital or between several of them. We can also find ways to assess doctors and hospitals using their digital avatars that are based on the information they give us, as well as “living” models. In the framework of all these fields, we built models that help doctors and administration understand and control the situation better,” explains Sergey Kovalchuk.
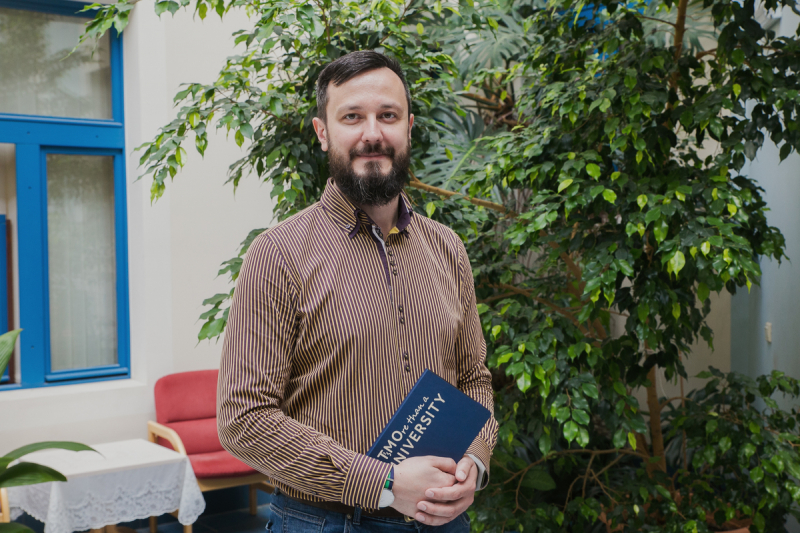
Sergey Kovalchuk
In order to modify the healthcare system, implement AI, and other high tech technologies, we need specialized methods and models. They not only allow us to evaluate the current situation or predict one but also optimize certain processes in medicine.
“Let’s say a hospital must decide what to fund: a new high tech equipment or a new department. What’s more beneficial? That’s where our models come in handy and allow us to understand various healthcare processes,” says Sergey Kovalchuk.
Another type of work performed by the lab’s researchers is organization, analysis, and integration of patient data. For example, chronic diseases develop slowly: this process takes many years and for the most part, the person lives their ordinary life without medical supervision.
The doctors don’t know what’s happening with the patient during this time but there are many factors that influence their state: habits, diet, sleeping patterns – these aspects often aren’t taken into consideration. However, if the patient uses a health app or a fitness tracker, it might be useful to collect data and choose appropriate treatment.
“By collecting this data and supplementing it with modeling, we can see the full picture. In order to integrate this system, we need both specialized modeling methods and tools for working with information. This is one of our areas of expertise,” says Sergey Kovalchuk.
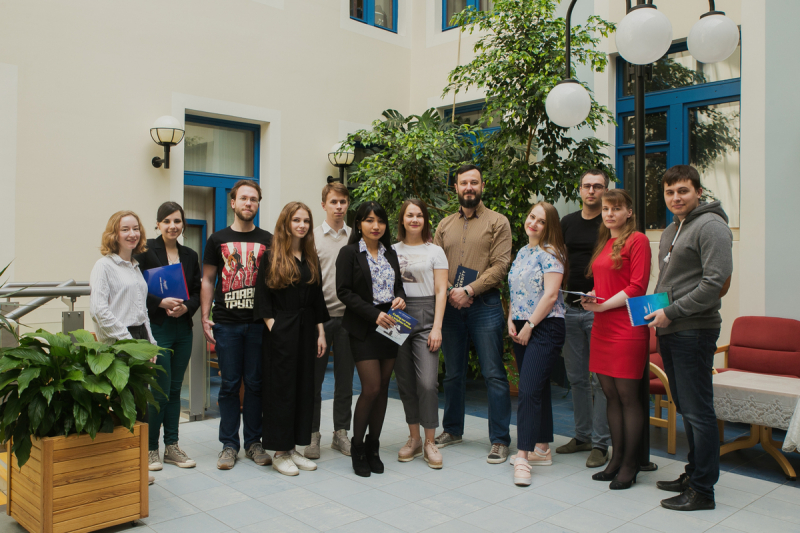
ITMO University’s Digital Healthcare Lab
What medical records say about patients
Most data in electronic medical recods is structured but some isn’t. In Russia, national medical information systems are now in development but the technological market and practice of using them are very diverse. This data can be structured with the help of medical text analysis.
“Take anamnesis, for instance. There are anamnesis vitae and anamnesis morbi: two groups of text that are barely structured from the machine’s point of view. Anamnesis vitae is what’s been happening with a patient, what kind of lifestyle they’ve had, what diseases they’ve had, etc. Anamnesis morbi is what has been happening with a person during the disease: how they’ve felt, what treatment they’ve received, and what the results are. These texts are written in a peculiar language that contains lots of abbreviations, incomplete sentences, and phrases in Latin. Usual text analysis tools won’t help here,” says Sergey Kovalchuk.
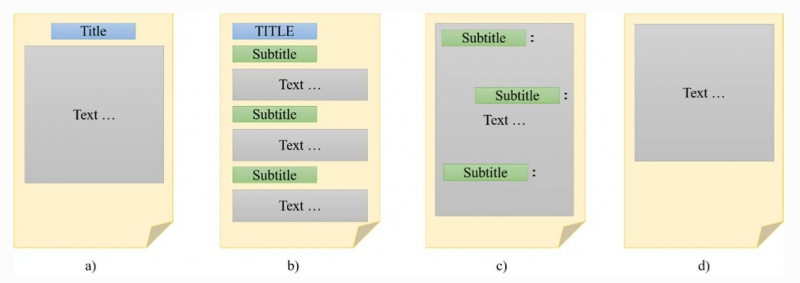
Different forms of unstructured textual EHR data. Credit: an article from the Applied Network Sciencehttps://appliednetsci.springeropen.com
In order to solve this problem, scientists extract genealogical anamnesis from the records, structurize symptoms and complaints, and formalize information. A similar project was developed by the lab jointly with the St. Petersburg Medical Information and Analytical Center.
“As a result, we can see how all these aspects influence the extent to which a city is information-based from technical to organizational features. Medical records are not only personalized in terms of patients, but also in terms of doctors – they write down the same things in different ways. Considering this, we need structuring and analytical tools for a range of tasks, from analysis of processes in a city to datasets that can be used for machine learning and prediction of bad outcomes,” says Sergey Kovalchuk.
Why analyze the behavior of patients and doctors
In order to build a model for a medical institution, you should establish the structure of all processes and behavior of all agents. This is called agent-based modeling.
“Agents (doctors, patients, nurses, and visitors) don’t act randomly – they have a certain hierarchy of goals. There are rules, according to which they solve their tasks; there’s a physical, informational, and logistical environment that they’re a part of. To see the big picture, we need to identify the rules and then implement them in the model, optimize them, and provide decision support,” says Sergey Kovalchuk.
As of now, the lab is working on a project together with the Almazov National Medical Research Centre. It’s about agent-based modeling and establishing trajectories and processes related to the doctors’ and patient’s activities in a hospital. Medical personnel are given carry-on labels to track their trajectories. Further, this might help make organizational decisions: for example, to optimize the timetable of staff or change the structure of departments.
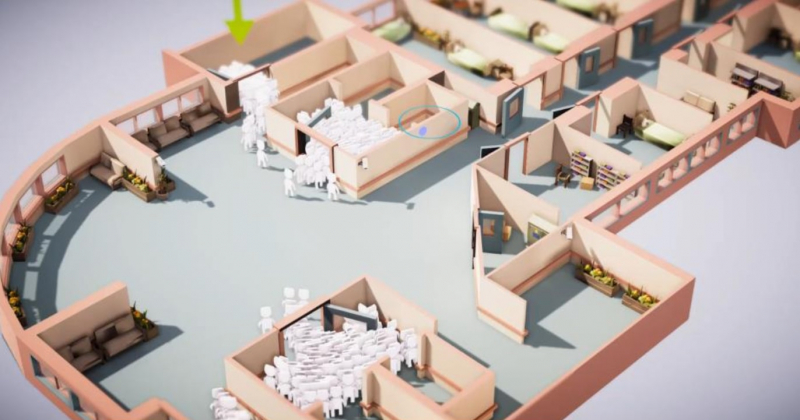
Agent-based model of a hospital
Decision support systems
Another field the researchers work in is the development of a decision support system that would help doctors make the right diagnosis.
“There are lots of decision support systems but they’re rarely used in practice because often the treatment they suggest isn’t compatible with the protocol a doctor follows. There’s a common joke that in the modern healthcare system, doctors don’t prescribe their patients with the most efficient treatment, but rather with the one that won’t be detected as the cause of death in case of an autopsy. Sadly enough, the doctor’s activities are highly regulated so decision support systems should take the protocols into consideration,” says Sergey Kovalchuk.
For example, a doctor has clinical recommendations on how to treat a patient – the system must take them into consideration. It’s not enough to simply offer a particular medication. The suggestion should be backed up by clinical research. However, not every doctor is ready to apply new technologies.
“When a decision support system is being implemented for testing, you can often observe that at first, the quality of medical services increase but then there is a setback. It’s a well-known thing and there are different, sometimes contradicting, explanations. For example, the doctors might’ve stopped using the system and ignored its recommendations. Vice versa, they might’ve started to follow them blindly, which isn’t good either as any algorithm can make mistakes. The system doesn’t make decisions, it supports them. It’s the doctor’s duty to trust the system and realize its benefits but at the same time, double-check its arguments and make the decisions themselves,” says Sergey Kovalchuk.
Modeling and enhancing the work of ambulances
At the Digital Healthcare lab, the specialists also research the modeling of dispatching and routing of ambulances. The goal is to optimize the process and help ambulances arrive at the spot sooner.
For example, if an ambulance is transporting a patient with acute coronary syndrome, they must reach the hospital as soon as possible. In St. Petersburg, there are about 15 places that are ready to accept such a patient. Each hospital has a certain number of operating rooms and they can be occupied by other urgent patients.
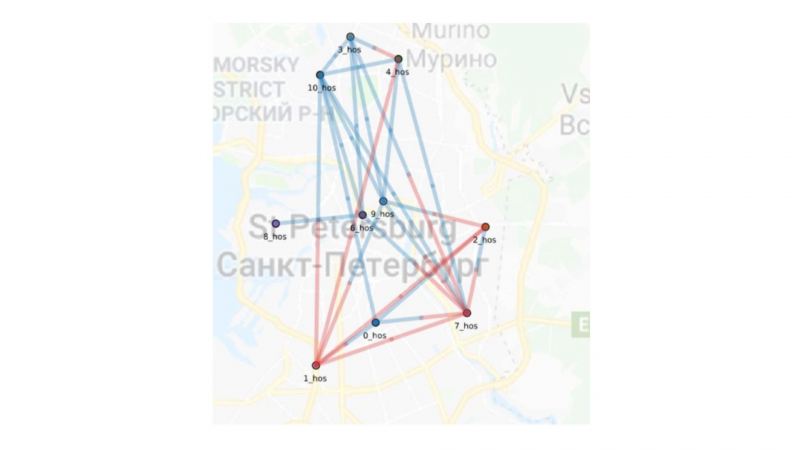
Determination of the current strategy for ambulance routes
So the ambulance workers must decide where to go – the nearest place might be occupied, and traveling to a far-away hospital is a big risk. Plus, the ambulance is always in a rush as well, as they have more calls waiting.
“How do you understand the behavior strategies of all agents and how do you manage them? Can we edit the existing patterns and thus decrease the death rate in a city? Models are the tool that helps us answer such questions before any real action has been taken,” says Sergey Kovalchuk.