Reasons to study gene expression
Gene expression is the process by which the information encoded in genes is used in the synthesis of proteins and other molecules crucial for cell functions. It helps researchers better understand the mechanisms underlying various diseases through analyzing the components involved in their development.
As of now, scientists have access to multiple databases that store information on gene expression, like Gene Expression Omnibus (GEO) and ArrayExpress. Nevertheless, single-cell sequencing data has been gaining increasing popularity, though such databases contain lots of unlabeled cells that cannot be identified without additional sample processing and a specialized expertise.
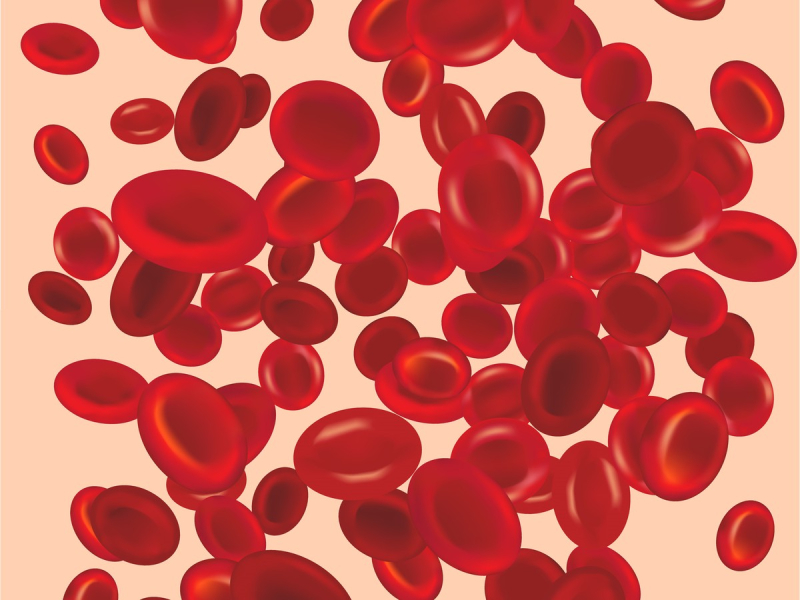
Credit: photogenica.ru
The dataset developed at ITMO
To systemize the available data, ITMO researchers and their colleagues from ImmGen (a group of labs working on the gene expression database for mouse immune system cells) and Tabula Muris (a project assembling a compendium of single-cell mouse transcriptome data, containing nearly 50,000 immune cells from 20 organs and tissues) designed a digital dataset for scientists to experiment with gene expressions of phagocyte genes using various mouse organs and form hypotheses about their potential applications. When developing the platform, the scientists identified all the studied cell types, thus making it easier to use for specialists from various fields.
The developed dataset is based on ImmGen Open Source, ImmGen Phase 1, and Tabula Muris Senis that provide information across 38 different tissues. For their research, the scientists studied three types of phagocytes: macrophages, dendritic cells, and monocytes. The cells examined are residents of the tissues they were taken from, meaning that they exhibit the same functions. Though hard to extract, these cells may manifest certain functions responsible for illnesses.
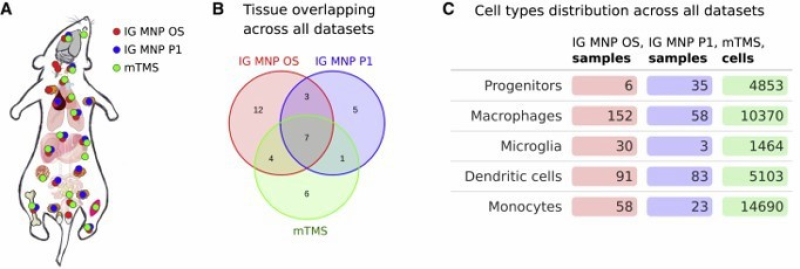
A tissue dataset used for sequencing: (A) Schematic illustration of tissue sampling; (B) Number of tissues overlapping across all datasets; (C) Cell-type distribution across all datasets. Illustrations from the article
Apart from the dataset, the researchers developed a method that allows users to describe big data associated with metabolism: splitting processes, utilization, and synthesis of different cell substances. With this method, called GAM-clustering, scientists can identify metabolic modules, which contain a co-regulated set of reactions needed to perform a particular cell function.
Suitable for both RNA and single-cell RNA sequencing, the approach makes it possible to deal with an infinitely large variety of cell states. While most bioinformatics methods compare cells from tissue A to those from tissue B, the method developed by the ITMO team can be used to analyze all the tissues and figure out metabolic features for each cell state, which was not possible for extensive data until now.
With the new method in hand, the researchers were able to identify the key metabolic features of various populations of phagocytic cells and thus analyze their correlations with cell types and their locations.
Some of the metabolic modules were verified experimentally, with the studies confirming the correctness of the GAM-clustering results. In particular, the acquired modules showed that in vivo interference with cholesterol synthesis reduces the migration abilities of dendritic cells, whereas glutathione, an intracellular antioxidant, is essential for the production of leukotrienes (a group of lipids) by peritoneal and lung macrophages.
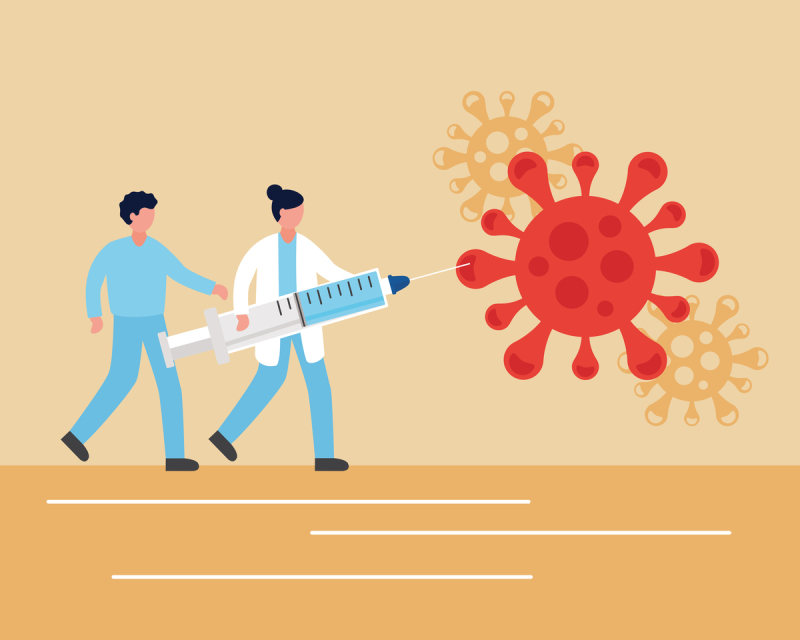
Credit: photogenica.ru
What lies ahead
“We need to know the difference between the metabolism of healthy and faulty cells. In that regard, scientists see an interference in macrophage metabolism as one of therapeutic strategies for treating and preventing certain diseases. Thus, macrophages play a decisive role in the development of atherosclerosis – a chronic condition in which cholesterol plaques build up in the walls of arteries. Macrophages that absorb so much cholesterol that they become dysfunctional are found to promote the formation of plaques. By interfering with their metabolism, we have the potential to prevent the development of the condition and even cure it,” says Anastasiia Gainullina, a co-author of the paper.
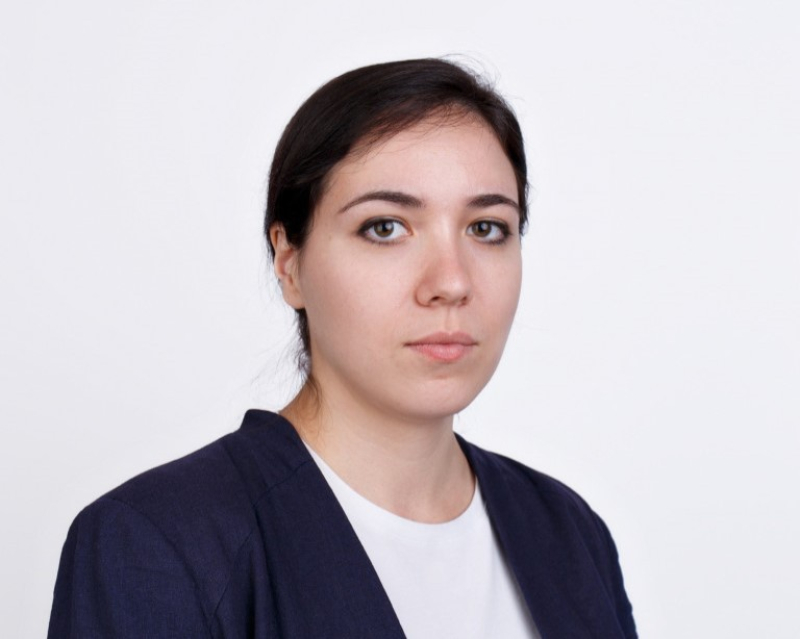
Anastasiia Gainullina. Photo by ITMO.NEWS
In case of cancer, macrophages exhibit both stimulating and inhibitory effects on a tumor. By modulating their metabolism, scientists can influence macrophage behavior and tumor progression. Even though the role of macrophages in different conditions is to be further studied, the progress so far feels pretty hopeful, notes Anastasiia Gainullina.
Reference: Anastasiia Gainullina, Denis Mogilenko, Li-Hao Huang, Helena Todorov, Vipin Narang, Ki-Wook Kim, Lim Sheau Yng, Andrew Kent, Baosen Jia, Kumba Seddu, Karen Krchma, Jun Wu, Karine Crozat, Elena Tomasello, Regine Dress, Peter See, Charlotte Scott, Sophie Gibbings, Geetika Bajpai, Jigar V. Desai, Barbara Maier, Sébastien This, Peter Wang, Stephanie Vargas Aguilar, Lucie Poupel, Sébastien Dussaud, Tyng-An Zhou, Veronique Angeli, J. Magarian Blander, Kyunghee Choi, Marc Dalod, Ivan Dzhagalov, Emmanuel L. Gautier, Claudia Jakubzick, Kory Lavine, Michail S. Lionakis, Helena Paidassi, Michael H. Sieweke, Florent Ginhoux, Martin Guilliams, Christophe Benoist, Miriam Merad, Gwendalyn J. Randolph, Alexey Sergushichev, Maxim Artyomov, ImmGen Consortium. Network analysis of large-scale ImmGen and Tabula Muris datasets highlights metabolic diversity of tissue mononuclear phagocytes (Cell Reports, 2023).