This year’s Nobel laureates are among the founders of the idea of neural networks. They have developed the architectures and training methods that made it possible to imitate human cognitive capacities for object detection and classification. The researchers approached this task from the perspective of statistical mechanics, drawing an analogy with magnetism and thermal motion.
“The laureates’ work has already been of the greatest benefit. In physics, we use artificial neural networks in a vast range of areas, such as developing new materials with specific properties,” says Ellen Moons, the Chair of the Nobel Committee for Physics.
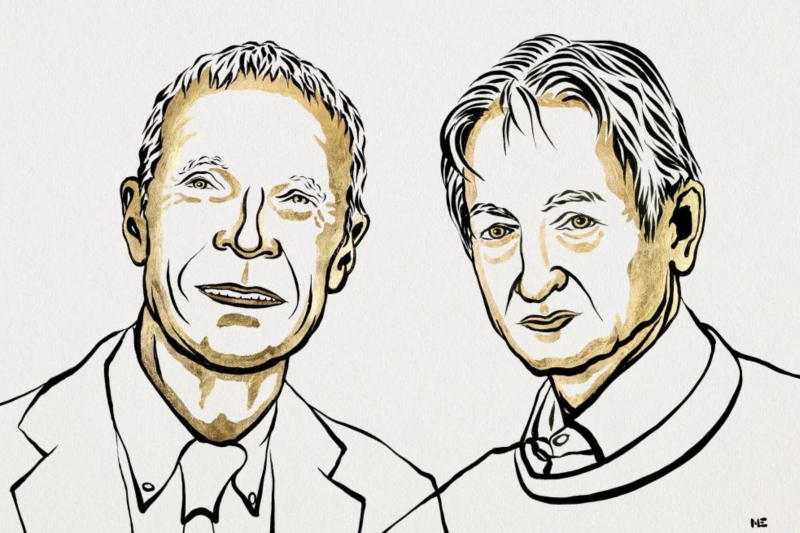
John J. Hopfield and Geoffrey E. Hinton. Image by Niklas Elmehed / Nobel Prize Outreach / www.nobelprize.org
Both researchers have been working with neural networks since the 1980s. In 1982, John J. Hopfield developed an associative neural network: a data processing model that can search a database for images that are similar, but not identical, to references. The idea behind the Hopfield network is based on statistical physics, meaning the correct organization of collective behavior of the network’s separate nodes.
In 1985, Geoffrey E. Hinton used the Hopfield network to develop a new data processing model based on the Boltzmann machine (named after Austrian physicist Ludwig Boltzmann, one of the founders of statistical mechanics). In contrast to the Hopfield network, Hinton’s model didn’t require references – it is capable of classifying images based on comparison, identifying common elements. In his work, Hinton also used statistical mechanics: the neural network is trained on the images that it will likely face in its work.
Through their work, Hinton and Hopfield brought neural networks closer to human thinking (as we don’t think in references, but rather compare one thing to another). That was a great leap towards the development of modern AI.
“The idea of the neural network is based on the functioning of the human brain – neurons connect to other neurons, creating complex neural connections. John Hopfield suggested for the signal to be transmitted from the output of each neuron in the network to the inputs of all other neurons. This architecture was one of the first networks capable of learning efficiently and quickly, as well as recovering damaged images. Geoffrey Hinton was one of the scientists who came up with the method of backpropagation, which is still used to train multilayer neural networks. Since the 1980s, neural networks have evolved significantly, with modern models consisting of trillions of neurons, but the technologies developed by Hopfield and Hinton remain as cornerstones of these systems,” shared Anton Kuznetsov, the head of ITMO’s Institute of Applied Computer Science.
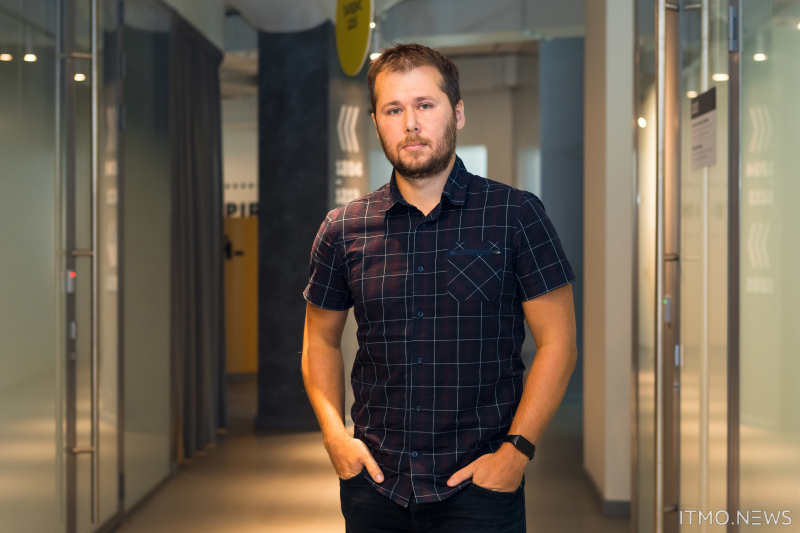
Anton Kuznetsov. Photo by Dmitry Grigoryev / ITMO.NEWS
According to Anton Kuznetsov, the laureates’ ideas are in use in all AI systems, from ChatGPT to generative models. For instance, ChatGPT is based on the idea of identifying similar objects.
Modern neural networks contain up to several trillion parameters and can solve a wide range of tasks: from generating images and music to identifying the specific disease that affects a patient. In these tasks, input data (such as images, text, and more) is transformed into sets of numbers and then fed into the neurons of the first layer. The signal then propagates through the subsequent layers, ultimately producing a result at the output. Naturally, a neural network cannot solve these tasks immediately; it needs to learn first – meaning it has to be provided with data for which the answer is already known and compare the resulting output with the reference. To minimize errors in the future, Geoffrey Hinton, along with a group of scientists, proposed the backpropagation method in 1986.
Hinton and Hopfield’s ideas also paved the way for those AI systems that are used in chemistry to predict chemical reactions – and in biology to develop next-gen treatments.
AI systems are also developed at ITMO. In 2024, a new lab, Generative Design of Enzymes and Aptamers, opened at the university; there, AI is applied to develop molecular machines: nanodevices that can catalyze chemical reactions, selectively bind molecules, and even act as biochips for diagnostics and therapy of various diseases. Recently, the researchers from ITMO’s ChemBio Cluster have created a web platform capable of predicting a nanozyme’s (artificial enzyme) capability to accelerate chemical reactions in mere seconds.