In some form, antibiotics are inevitably used for treatment or prevention of diseases in farm animals – and thus traces of antibiotics in products of animal origin present a serious issue for consumers and producers alike. They can have various negative effects on human health, such as cause allergies or lead to antibiotic resistance. That’s why their presence in food products is strictly regulated by states and retailers, meaning that farmers and suppliers are obligated to test their products before they end up on store shelves.
It’s common practice to use test strips to analyze dairy products; however, their accuracy rarely exceeds 70% and the method itself is limited to detecting only the presence of antibiotics, not their quantity. Scientists from ITMO’s Infochemistry Scientific Center have suggested a new, more reliable technology based on electrochemical analysis and machine learning; it consists of three parts: an electrode sensor, a potentiostat, and ML-based software.
“First, we placed a test sample onto an electrode sensor made from copper, nickel, and carbon – substances that oxidize when they come into contact with milk. Next, using the potentiostat (a device that can produce the current of a given voltage) we ran the current through the electrode. The potentiostat was also instrumental in measuring the sensor’s response: when a metal is oxidized, its conductivity decreases – and the potentiostat registers this as voltage changes. The fewer antibiotics in the sample, the more the metal in the electrode is oxidized by the lactic acid created by bacteria. Consequently, the voltage in the entire system decreases,” explains Vadim Belyaev, one of the technology’s developers and a PhD student at ITMO’s Infochemistry Center.
The research team has conducted a series of experiments with the five most common antibiotics: streptomycin, penicillin, tetracycline, cefazolin, and ceftiofur (the latter has only veterinary applications). Milk powder solutions with different antibiotics and their combinations interacted differently with the electrode’s surface; the results of these reactions could be seen as spikes in current-voltage features. All the data collected during these experiments was assembled into a single dataset to train an ML algorithm, which, as a result, can analyze incoming signals from the electrode and potentiostat to automatically determine the amount of antibiotics in a sample.
One of the project’s industrial partners is Galaktika Group, a major dairy producer. According to the researchers, their technology can be adapted for various purposes. For instance, in collaboration with Gazpromneft they used a similar approach to develop a device for detecting unwanted contaminants in oil. The scientists have also suggested using this method to optimize detection of antioxidants in wine, as well as the kinds of grapes used to make it and the location they originated from.
In its current state, the system will have to be significantly reconfigured to be fit for other applications: it will require a different metal composition of the electrode and a new library of samples for the algorithm. In the near future, the research team is planning to automate the process of dataset creation with the most advanced ML models.
“We are currently trying to generate data using various software, such as COMSOL Multiphysics. My biggest dream, however, is to develop a variational autoencoder and transformer that will be able to function exclusively on synthetic data by the time I complete my PhD. This way, we won’t need to perform manual calculations of, say, zinc in water or corrosion inhibitors in oil. We want to make a technology that will be easy to use and capable of learning and adapting to new tasks,” concludes Timur Aliev, the paper’s first author and a PhD student at ITMO’s Infochemistry Center.
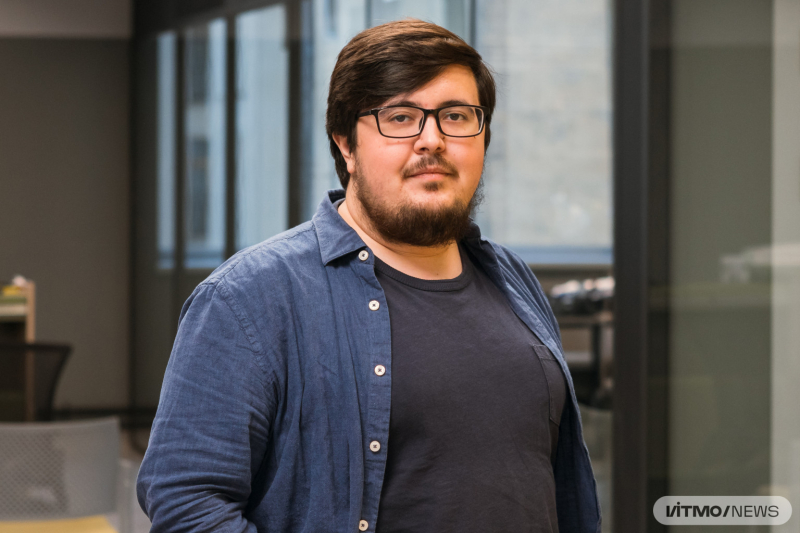
Timur Aliev. Photo by Dmitry Grigoryev / ITMO.NEWS
This project is supported by the Russian Science Foundation (Project No. 23-16-00224).
Reference: Timur Aliev, Vadim Belyaev, Anastasiya Pomytkina, Pavel Nesterov, Sergey Shityakov, Roman Sadovnichii, Alexander Novikov, Olga Orlova, Maria Masalovich, Ekaterina Skorb, Electrochemical Sensor to Detect Antibiotics in Milk Based on Machine Learning Algorithms. ACS Applied Materials & Interfaces, 2023.