Magnetic heterostructured nanoparticles are no more than 100 nanometers in size, and their shell and core can act as nanomagnets and attract other magnetic materials. They can also serve as electrical conductors and information “carriers,” such as in hard drives. Due to these properties, such nanoparticles are widely used in the production of electronic devices, including the most precise microelectronics, as coatings for various parts that enhance their electromagnetic and electronic characteristics. At the same time, in order for the particles to be used, they must exhibit certain magnetization “parameters.” These can be controlled using four key properties of magnetic nanoparticles, one of which is exchange bias. Until now, its prediction required complex mathematical calculations and modeling, but the ML model trained by ITMO scientists is capable of predicting the magnitude of the exchange shift in just a couple of minutes.
In order to train the model, the team manually assembled over 1,000 lines of data. Two ML models were chosen to test out the method: the convenient XGBoost (2014) and the network-based and popular among researchers KAN (2024). In the course of experiments, the first model proved to be more efficient, explaining 75% of variations in data, which is quite a high level of accuracy. To verify their results, the researchers compared the data produced by the model to those published in nanoparticle research; they also relied on cross-validation.
“Our model predicts the magnitude of the exchange shift based on more than 30 nanoparticle parameters, among which are their shape and size, temperature dependencies, coercive power, magnetic field strength, and others. You simply input these parameters into the code, and the model will predict whether an exchange shift will occur in these particles, and if so, its magnitude. This way, you don’t need to run complex calculations and experiments with highly sensitive nanoparticles in order to find the ones best suited for a given purpose,” says Ksenia Kapranova, the author of the project and a Master’s student at ITMO’s Center for AI in Chemistry (ChemBio Cluster).
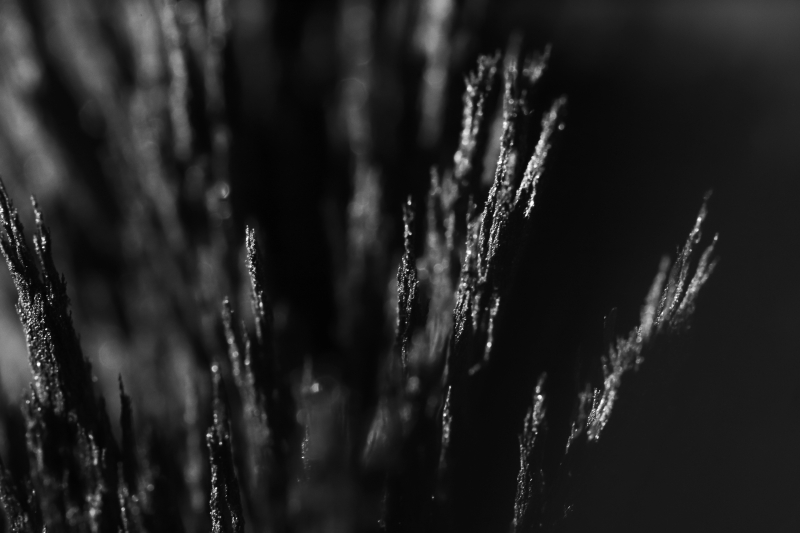
Credit: deniskvarda / photogenica.ru
This is the first time in scientific history that such a technology is used to predict the exchange shift. However, earlier, a team from ITMO has already used an ML model to predict the form and size of nanoparticles, as well as some of their magnetic properties – for instance, their coercive power, which may be used to increase the precision of MRI units.
“Our technology opens new avenues for materials science. We have laid the groundwork for a platform that will be able to account for more parameters, including nanoparticle synthesis. We will be able to predict the materials necessary to synthesize particles with desired parameters, thus significantly reducing the number of experiments and conducting more targeted research based on the model’s output. This, in turn, will improve the efficiency of chemical laboratories and time and resources,” adds Daniil Kladko, the head of the project and a researcher at ITMO’s Center for AI in Chemistry (ChemBio Cluster).
The research project was conducted with support from the Russian Science Foundation within the national program Priority 2030.