It all starts with chemometrics
The testing device was first developed in 2020, when researchers from ITMO’s Infochemistry Scientific Center tested its ability to detect tick-borne encephalitis in a biological sample. Today’s version of the device is a significantly improved one, although based on the same analysis principle, and is now used to detect bacteria in dairy products.
In order to be analyzed, a sample has to be mixed into a special agar-based hydrogel that contains electrolytes. Next, liquid hydrogel is poured into an electrochemical cell, where it is left to solidify. The cell contains electrodes made of a liquid eutectic alloy of gallium and indium. Then, electrical current in different voltage ranges runs through the electrodes, which touch the hydrogel, while the connected potentiostat registers the current’s fluctuations. This data is visualized as volt-ampere curves and analyzed by a specially trained machine learning algorithm.
According to Master’s student Filipp Lavrentev, one of the developers of the device, current fluctuations are caused by reduction-oxidation reactions in metabolites produced by bacteria. For instance, lactic acid causes the oxidation of gallium in the electrochemical cell, which is registered by the potentiostat.
“The secret to our device is the special interaction between hydrogel and the liquid alloy, which would be impossible with regular solid electrodes. When the electrodes and the hydrogel are subjected to electrical current, chemical interactions occur at their borders, enveloping the electrode in a layer of gallium lactate. Depending on the amount of bacteria in the hydrogel and consequently the metabolite they produce, the thickness of the film increases and changes the resistance,” explains Filipp Lavrentev.
Spikes in volt-ampere curves can be used to estimate the concentration of bacteria based on the amount of their metabolite (lactic acid) that they produce.
Machine learning vs manual computations
All calculations are done by the multilayer perceptron-based machine learning algorithm that was specifically trained for this purpose. Its input data are current fluctuations in various resistance ranges.
The algorithm’s accuracy is 94%, and it currently works with only two types of bacteria: Streptococcus thermophilus and Bacillus coagulans. Importantly, a new algorithm has to be written for each new task – and a large amount of data has to be collected to train it. For example, a group of researchers is now accumulating a database that will be used to detect antibiotics in milk as part of a commercial collaboration with an industrial partner.
Not only scientists, but also school students are engaged in the development thanks to their internship at the Infochemistry Scientific Center as part of the Sirius.Summer initiative. For instance, students under the supervision of Filipp Lavrentev are working on the project for detecting intestinal bacterium Escherichia coli.
Collaboration with the industry
Conventionally, bacteria are detected with a highly accurate yet extremely time-consuming method. Researchers plant a bacterial culture on a breeding ground and place it inside a thermostat, and then they have to wait for the bacteria to grow – typically for up to 72 hours. Finally, the bacteria are manually counted in their dishes by highly qualified specialists and in completely sterile conditions.
With the newly-developed method, researchers won’t have to manually count anything and will have the result ready in only 15-20 minutes. However, Filipp Lavrentev admits that despite the palpable interest of industry representatives (experts from Danone and the Galactica Group Group have already visited the lab), there are still a number of challenges to overcome before the device can be introduced into production. They mostly have to do with certification and compliance with the existing state standards.
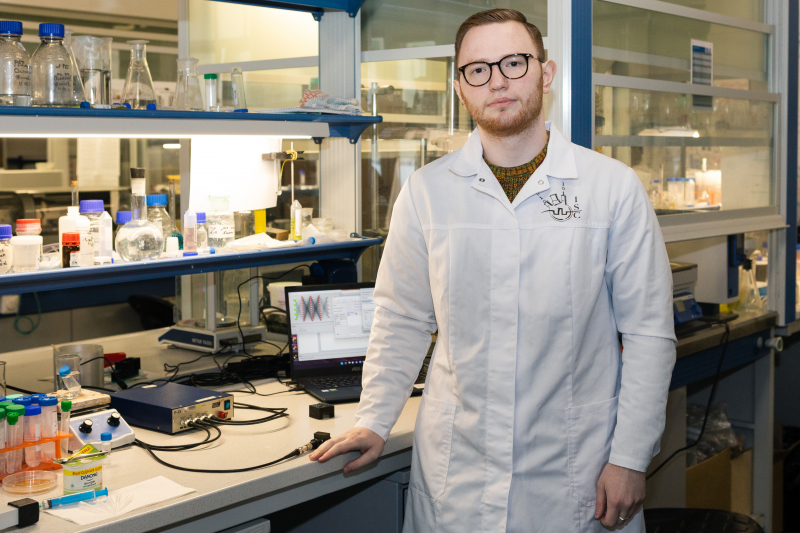
Filipp Lavrentev. Photo by Dmitry Grigoryev, ITMO.NEWS
On another note, the device also has the potential to be applied for another purpose – the analysis of oil derivatives. According to the researchers, the system can be adapted to analyze any type of liquid. In the case of oil products, one would only need to switch the compounds of the gel and use a polar solvent instead of water due to the hydrophobic qualities of oil.
To make the device more convenient and user-friendly, the researchers are planning to eventually replace the bulky set-up of the potentiostat, the testing platform, and the computer into one compact unit with a simple interface that will display the results of the analysis.
Hypothetically, the device could be used not only in production, but also for at-home blood and saliva tests. Moreover, given that hydrogel is completely biocompatible, in the distant future this way of testing could even be used for internal monitoring of the human body.
Reference: Filipp Lavrentev, Igor Rumyantsev, Artemii Ivanov, Vladimir Shilovskikh, Olga Orlova, Konstantin Nikolaev, Daria Andreeva, and Ekaterina Skorb. Soft Hydrogel Actuator for Fast Machine-Learning-Assisted Bacteria Detection. ACS Applied Materials & Interfaces, 2022.