Why scan for toxicity
AI and machine learning are successfully applied in organic chemistry, for instance, to predict the 3D structure of proteins and the properties of materials, or to develop drugs. However, employing these methods for nanomaterials has its challenges – for one, it is much harder to describe nanosystems in a form recognizable by algorithms. That’s why, whereas algorithmic prediction of physical (optical, magnetic, etc.) properties of nanomaterials can be quite accurate, predicting their biological behavior remains a challenge.
As nanomaterials are actively applied in medicine for diagnostics and in targeted drug delivery systems, predicting their biological properties becomes highly important: if the materials interacting with living tissues prove to be toxic, they can cause great damage. Therefore, in order to develop a safe drug delivery system, all the materials involved have to be tested for toxicity.
The current machine learning models are binary, offering a yes or no answer to the question. However, they do not account for potential effects caused by higher concentration of a given material – and this information is crucial for researchers whose job is to determine the security of nanomaterials.
What ITMO chemists suggest
A new approach to quantitative prediction of inorganic nanomaterial cellular toxicity was suggested by a team from ITMO, featuring school (Ilya Petrov, Yurii Seregin) and university students (Nikolai Shirokii, Yevgeniya Din, Sofia Sirotenko, Julia Razlivina), headed by PhD student at ChemBio Cluster Nikita Serov and head of the cluster Vladimir Vinogradov. Their method is based on machine learning algorithms and is capable of reproducing concentration dependencies typically obtained via experiments.
After 10-fold cross-validation, the algorithm’s error rate was 12%, which is considered to be a good result. The algorithm is capable of predicting material toxicity with precision that won’t affect the dynamics of respective chemical processes.
Applications of the algorithm
Among the new algorithm’s potential applications is screening of nanomaterials involved in cancer diagnostics and treatments for safety and toxicity. Apart from serving as drug delivery systems, nanomaterials can serve as contrasts, helping to detect tumors during MRI scanning. It is crucial to test such nanomaterials before introducing them into actual practice.
From a datacon to a publication
The team came up with the algorithm at DataCon, an event held by ITMO’s Center for Artificial Intelligence in Chemistry in the summer of 2022. There, participants performed all things data: collecting, cleaning, visualization, doing statistical and graphical analysis; they also screened models to select the best one and validated its result on different systems. Each team had to upload their solution to GitHub. As a result of the hackathon, the toxicity prediction algorithm achieved the highest performance metrics.
“At the hackathon, we received five datasets from real-life cellular toxicity tests of nanomaterials. Each dataset contained descriptions of nanomaterials (their chemical compounds, particle diameters, surface area and charge), as well as experimental parameters: particle concentration, timing, and cell line characteristics. We compiled all datasets into one and prepared it for ML processing by checking for unwanted correlations and outliers. We decided to use gradient boosting (CatBoostRegressor) to build a regression model of cell survival after interaction with nanomaterials. This method consecutively forms ensembles of weaker models, such as decision trees. Having developed a working algorithm, we tested it by visualizing the parameters that determine its decisions,” explained Yevgeniya Din, a member of the team and a Bachelor’s student at ITMO.
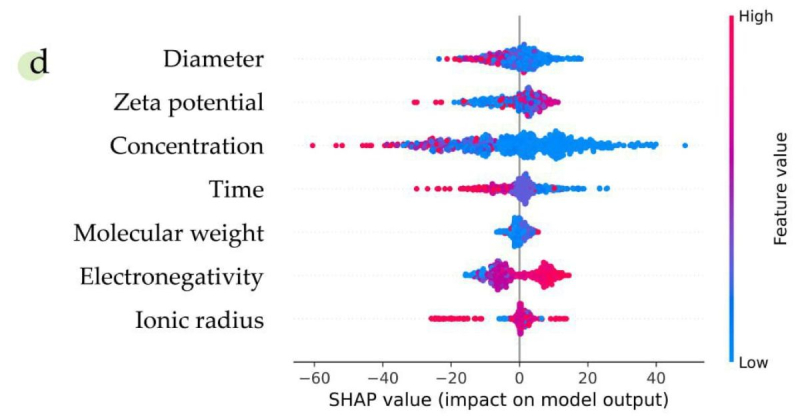
The algorithm follows experimental logic when producing its solutions, taking into account the way each parameter affects the resulting toxicity. Visualizations courtesy of the paper’s authors
What’s next
The researchers are now planning to integrate their algorithm with others developed at the Center for Artifical Intelligence in Chemistry, thus assembling a larger project in the form of a website that will predict various properties of nanomaterials. An inspiration behind this initiative is Materials Project, an open-source website that calculates the properties of materials for physical and other applications. One goal pursued by the researchers is making such solutions more accessible to experimental scientists.
“Our current objective is smoothly integrating this algorithm into other solutions so that we can predict such target indicators as catalytic activity, delivery efficiency, as well as materials’ safety. Testing for safety is important, because sometimes algorithms can suggest a solution that will kill 100% of cell lines at its lowest concentration. We are planning to develop an open-source website for the scientific community, where we will present a clean dataset that others will be able to use in their solutions. We believe that experiments have to be valid and reproducible, which is why we argue that such platforms have to be open to the public,” said Nikita Serov, one of the supervisors of the project.
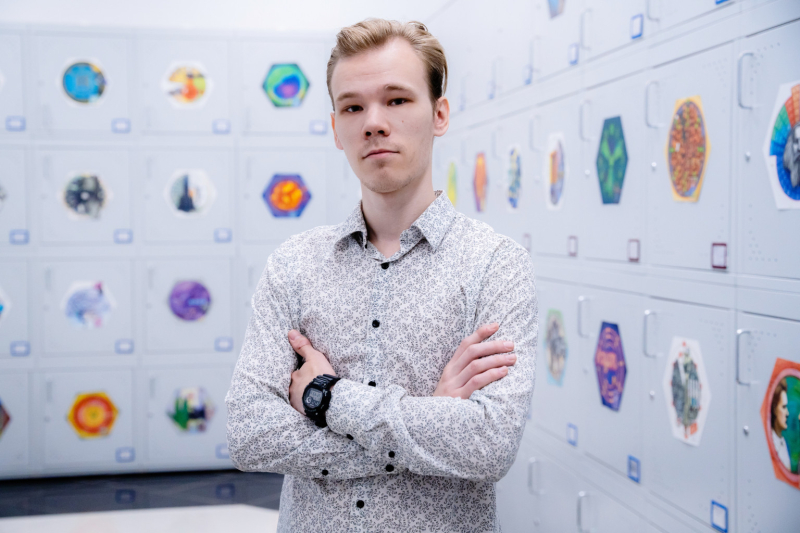
Nikita Serov. Photo by Margarita Erukova / ITMO.NEWS
Reference: Nikolai Shirokii, Yevgeniya Din, Ilya Petrov, Yurii Seregin, Sofia Sirotenko, Julia Razlivina, Nikita Serov, Vladimir Vinogradov. Quantitative Prediction of Inorganic Nanomaterial Cellular Toxicity via Machine Learning (Small, 2023)